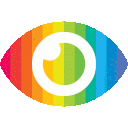
1. Lithium-ion batteries require effective management to extend service life and increase reliability and safety.
2. The state of health (SOH) of batteries can be estimated using experimental, model-based, and machine learning methods.
3. Internal resistance measurement is a vital part of SOH assessment, but it is influenced by various factors such as battery capacity, temperature, and discharge rate.
The article titled "State of Health Estimation Methods for Lithium-Ion Batteries" provides a comprehensive review of the various methods used to estimate the state of health (SOH) of lithium-ion batteries. The article highlights the importance of accurate SOH estimation in predicting battery life, preventing potential damage, and enhancing performance.
The article discusses three main methods for estimating SOH: experimental, model-based, and machine learning methods. While each method has its pros and cons, the article concludes that experimental methods are not applicable for commercial cases due to their time-consuming nature and potential for systematic errors.
One potential bias in the article is its focus on model-based and machine learning methods over experimental methods. While it is true that experimental methods can be time-consuming and prone to errors, they still play an essential role in understanding battery aging behavior. Additionally, the article does not provide a balanced discussion of the advantages and disadvantages of each method.
Another potential bias is the lack of consideration given to external factors that can affect battery performance, such as environmental conditions and usage patterns. While internal factors like aging and temperature are discussed extensively, external factors can also have a significant impact on battery life.
The article also makes unsupported claims about the accuracy of SOH estimation using machine learning methods. While these methods have shown promise in predicting battery life based on past data, there is still much research needed to validate their effectiveness in real-world applications.
Overall, while the article provides a useful overview of SOH estimation methods for lithium-ion batteries, it could benefit from a more balanced discussion of each method's advantages and disadvantages and greater consideration given to external factors that can affect battery performance.