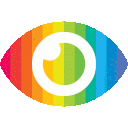
1. Lithium-ion batteries are widely used in various applications, and the state of health (SOH) estimation is crucial for their performance, safety, and reliability.
2. Data-driven methods using machine learning models have become a research hotspot for SOH estimation, with various health indicators (HIs) extracted from charging or discharging curves or electrochemical impedance spectroscopy (EIS).
3. A proposed constant current charging time (CCCT) based SOH estimation method using random forest regression (RFR) algorithm achieves higher accuracy with less data and features, simpler model structure, and faster training and estimation speed. The method also corresponds direct features from the charging curve to IC peak for better results.
The article titled "Constant current charging time based fast state-of-health estimation for lithium-ion batteries" provides an overview of the various methods used to estimate the state of health (SOH) of lithium-ion batteries. The article highlights the importance of accurate SOH estimation in ensuring the performance, safety, and reliability of batteries in various applications.
The article discusses three categories of SOH estimation methods: direct measurement, model-based methods, and data-driven methods. The data-driven method is presented as a research hotspot due to its flexibility and universality. The article also provides examples of studies that have used machine learning models such as Gaussian process regression (GPR), support vector machine (SVM), random forest (RF), and neural network (NN) to estimate SOH.
The article then focuses on the limitations of current data-driven methods, including difficulties in obtaining selected features to characterize battery degradation, complex model structures, long training times, and large charging voltage segments. The article proposes a new method based on constant current charging time (CCCT) and random forest regression (RFR) algorithm that uses less data and features with a simpler model structure to achieve higher SOH estimation accuracy.
Overall, the article provides a comprehensive overview of SOH estimation methods for lithium-ion batteries. However, there are some potential biases in the article. For example, the article focuses primarily on data-driven methods while downplaying other categories such as direct measurement and model-based methods. Additionally, while the proposed CCCT-based method is presented as a solution to current limitations in data-driven methods, there is no discussion or comparison with other potential solutions.
Furthermore, while the article acknowledges some limitations of previous studies such as difficulties in obtaining selected features and complex model structures, it does not explore potential counterarguments or alternative perspectives on these issues. Additionally, there is no discussion on possible risks associated with using machine learning models for SOH estimation or how these risks can be mitigated.
In conclusion, while the article provides valuable insights into SOH estimation for lithium-ion batteries and proposes a new method based on CCCT and RFR algorithm that addresses some limitations of current data-driven methods, it could benefit from more balanced reporting that considers alternative perspectives and potential risks associated with using machine learning models for SOH estimation.