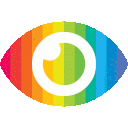
1. Lithium-ion batteries are widely used in various industries due to their high energy density, power density, and longer cycle time.
2. Accurately predicting the state of health (SOH) of lithium-ion batteries is crucial for their secure operation and assessing degradation and aging mechanisms.
3. The proposed CNN-BiLSTM-AM model integrates the merits of CNN, BiLSTM, and attention mechanism (AM) to accurately predict the SOH of lithium-ion batteries by extracting features from time series data and emphasizing correlation and long-term dependencies.
The article "State-of-Health Prediction of Lithium-Ion Batteries Based on CNN-BiLSTM-AM" provides an overview of the methods used for predicting the state of health (SOH) of lithium-ion batteries. The article discusses the limitations of model-based methods and highlights the advantages of data-driven approaches, particularly deep learning models such as recurrent neural networks (RNNs), long- and short-term memory networks (LSTMs), and hybrid models that combine different types of methods.
The article presents a new model called CNN-BiLSTM-AM, which combines convolutional neural networks (CNNs) with bidirectional LSTMs and attention mechanisms (AMs) to predict SOH accurately. The authors explain how CNNs extract local features from time series data, while BiLSTMs capture forward and backward dependencies to emphasize the correlation between time series data and capture long-term dependencies. The AM is used to weight the time series data associated with SOH, resulting in more accurate predictions.
Overall, the article provides a comprehensive overview of the current state-of-the-art in SOH prediction for lithium-ion batteries. However, there are some potential biases and limitations to consider. For example, the article focuses primarily on deep learning models and does not discuss other types of machine learning algorithms or statistical models that may be useful for predicting SOH. Additionally, while the authors mention some limitations of CNNs, they do not provide a detailed analysis of these limitations or explore alternative approaches to address them.
Another potential limitation is that the article focuses on a specific dataset from NASA without discussing how well their proposed model would generalize to other datasets or real-world applications. It would be helpful if future research could evaluate the performance of this model on different datasets or compare it with other existing models.
In conclusion, while this article provides valuable insights into predicting SOH for lithium-ion batteries using deep learning models, it is important to consider its potential biases and limitations when interpreting its findings. Future research should continue to explore alternative approaches and evaluate their performance on different datasets to improve our understanding of battery degradation mechanisms and optimize battery design and management.