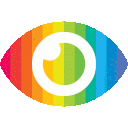
1. Accurate state of health (SOH) estimation of lithium-ion batteries is crucial for reliable and safe operations of electric vehicles.
2. A hybrid attention and deep learning method is proposed for SOH prediction, which extracts health features related to capacity degradation from differential temperature curves and integrates the strengths of convolutional neural network, gated recurrent unit recurrent neural network, and attention mechanism.
3. The proposed method outperforms eleven mainstream methods in terms of prediction performance, with all estimation errors maintained within 1.3% without extracting highly correlated health features, demonstrating promising accuracy and reliability.
The article titled "State of Health Estimation for Lithium-ion Batteries Based on Hybrid Attention and Deep Learning" presents a new method for predicting the state of health (SOH) of lithium-ion batteries using a hybrid attention and deep learning approach. The authors argue that accurate SOH estimation is crucial for ensuring the safe and reliable operation of electric vehicles, which rely on these batteries as their primary power source.
The article provides a comprehensive overview of existing methods for SOH estimation, including experiment-based, model-based, and data-driven approaches. The authors note that while each of these methods has its strengths and weaknesses, data-driven methods have gained widespread attention due to their ability to directly build SOH prediction models from historical battery data.
The proposed method involves calculating temperature difference curves from charging data, smoothing them with a Kalman filter, extracting health features related to capacity degradation from the differential temperature curves, and using a hybrid attention and deep learning model to forecast the battery's SOH. The authors claim that this approach outperforms eleven mainstream methods in terms of prediction accuracy.
While the article provides a detailed description of the proposed method and its advantages over existing approaches, it does not explore potential limitations or drawbacks. For example, it is unclear how well this method would perform under different environmental or loading conditions or whether it could be applied to other types of batteries beyond lithium-ion.
Additionally, the article does not provide any information about potential biases or conflicts of interest that may have influenced the research findings. It is possible that the authors have a vested interest in promoting this particular approach over others or that they may have overlooked certain limitations or challenges in their analysis.
Overall, while the proposed method appears promising based on the results presented in this article, further research is needed to fully evaluate its effectiveness and applicability in real-world settings. Additionally, more transparency around potential biases or limitations would help readers better understand the implications of these findings.