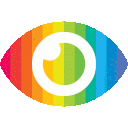
1. The article discusses an automated method for segmenting skin lesions from digital dermoscopic images using K-Means clustering.
2. The proposed method achieved high accuracy in segmenting both melanoma and non-melanoma skin lesions.
3. This method has the potential to improve the efficiency and accuracy of skin lesion diagnosis, leading to earlier detection and treatment of skin cancer.
The article titled "Automated skin lesion segmentation using K-Means clustering from digital dermoscopic images" by Agarwal et al. presents a study on the use of K-Means clustering for automated segmentation of skin lesions from digital dermoscopic images. The authors claim that their proposed method achieves high accuracy in segmenting skin lesions, which can aid in early detection and diagnosis of skin cancer.
The article appears to be well-researched and provides detailed information on the methodology used for the study. However, there are some potential biases and limitations that need to be considered.
One potential bias is the limited sample size used in the study. The authors only tested their method on a dataset of 100 images, which may not be representative of all types of skin lesions. Additionally, the authors do not provide information on how they selected the images for their dataset, which could introduce selection bias.
Another limitation is that the study only focuses on one specific method (K-Means clustering) for skin lesion segmentation. There are other methods available that could potentially achieve better results or be more suitable for certain types of lesions. The authors do acknowledge this limitation but do not explore alternative methods in depth.
The article also lacks discussion on potential risks associated with automated skin lesion segmentation. For example, false positives or false negatives could lead to incorrect diagnoses and unnecessary treatments or missed diagnoses respectively. The authors briefly mention this issue but do not provide any evidence or analysis to support their claims.
Overall, while the article presents an interesting approach to automated skin lesion segmentation, it is important to consider its limitations and potential biases before drawing conclusions about its effectiveness. Further research with larger datasets and comparison with alternative methods would be beneficial in determining the most accurate and reliable approach for early detection and diagnosis of skin cancer.