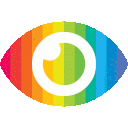
1. Rare diseases have extremely low-data regimes, making it challenging to train a neural network to classify them with few per-class data samples.
2. A difficulty-aware meta-learning method was developed to address rare disease classifications and demonstrated its capability to classify dermoscopy images.
3. The method dynamically monitors the importance of learning tasks during the meta-optimization stage and can quickly adapt to classify unseen classes with only five samples per class.
The article titled "Difficulty-Aware Meta-learning for Rare Disease Diagnosis" presents a new method to address the challenge of classifying rare diseases with limited data. The authors propose a difficulty-aware meta-learning approach that adapts a pre-trained model on common diseases to perform rare disease classification. The study demonstrates the effectiveness of this approach in classifying dermoscopy images and achieving high AUC scores.
Overall, the article provides valuable insights into the challenges of diagnosing rare diseases and proposes an innovative solution to address this issue. However, there are some potential biases and limitations in the study that need to be considered.
One limitation is that the study only evaluates the proposed method on skin lesion classification using the ISIC 2018 dataset. While this is a relevant application area, it would be beneficial to test the method on other types of rare diseases and datasets to assess its generalizability.
Another potential bias is that the study focuses solely on deep learning approaches and does not consider other machine learning techniques or hybrid models. This could limit the scope of potential solutions for addressing rare disease diagnosis.
Additionally, while the article mentions real clinical practice as a potential application area for their method, there is no discussion of any potential risks or limitations associated with using AI-based systems for medical diagnosis. It would be important to consider ethical concerns such as patient privacy, accuracy, and accountability when implementing such systems in clinical settings.
Furthermore, while the article presents evidence supporting their claims about the effectiveness of their proposed method, there are no counterarguments or alternative perspectives presented. This could lead to one-sided reporting and limit critical evaluation of their approach.
In conclusion, while the article presents an innovative solution for addressing rare disease diagnosis using difficulty-aware meta-learning, it is important to consider potential biases and limitations in their study. Further research is needed to evaluate its generalizability across different types of rare diseases and datasets and assess its practical implications in real clinical settings.