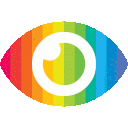
1. MetaMed is a few-shot learning approach for medical image classification that can adapt to rare disease classes with the availability of few images and less compute.
2. The model uses advanced image augmentation techniques like CutOut, MixUp, and CutMix to overcome the problem of over-fitting and increase generalization capability by 2-5%.
3. MetaMed outperforms transfer learning for 3, 5, and 10-shot tasks for both 2-way and 3-way classification on three publicly accessible medical datasets – Pap smear, BreakHis, and ISIC 2018.
The article "MetaMed: Few-shot medical image classification using gradient-based meta-learning" discusses the challenges of using conventional deep learning techniques for medical image classification due to the scarcity of high-quality annotated images and long-tailed distributions. The authors propose a few-shot learning problem and present a meta-learning-based approach called MetaMed, which can adapt to rare disease classes with the availability of few images and less compute. The model is validated on three publicly accessible medical datasets – Pap smear, BreakHis, and ISIC 2018.
The article provides a detailed overview of the motivation behind the proposed approach and its potential contributions to the field of medical image classification. However, there are some potential biases in the article that need to be considered. For example, the authors do not discuss any limitations or drawbacks of their proposed approach, which could lead readers to believe that it is a perfect solution for medical image classification.
Additionally, while the authors mention that advanced augmentation techniques like CutOut, MixUp, and CutMix were used to overcome over-fitting problems, they do not provide any evidence or data to support this claim. Furthermore, there is no discussion about how these techniques were chosen or why they were effective.
The article also lacks a thorough analysis of potential risks associated with using MetaMed for medical image classification. For example, there may be ethical concerns related to relying solely on AI models for diagnosis without human oversight.
Overall, while the article presents an interesting approach for medical image classification using meta-learning techniques, it would benefit from more balanced reporting that acknowledges potential limitations and risks associated with this approach.