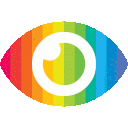
1. Machine learning, including deep neural networks, is being used in stroke rehabilitation to predict recovery of motor function and interpret medical imaging data.
2. Commonly used machine learning models in stroke research include random forest, logistic regression, and deep neural networks.
3. Machine learning has the potential to be used for various purposes in stroke rehabilitation, such as predicting the occurrence of depression and the recovery of language, cognitive, and sensory function.
The article titled "Use of Machine Learning in Stroke Rehabilitation: A Narrative Review" provides an overview of the applications and research on machine learning in stroke rehabilitation. While the article offers valuable insights into the potential use of machine learning in predicting motor function recovery and interpreting medical imaging data, there are several areas where critical analysis is warranted.
One potential bias in the article is the limited focus on specific machine learning models, such as random forest, logistic regression, and deep neural networks. While these models have been commonly used in medical research, there are other machine learning algorithms that could also be relevant to stroke rehabilitation. The article does not explore alternative models or discuss their potential advantages or limitations.
Additionally, the article lacks a comprehensive discussion of the limitations and challenges associated with using machine learning in stroke rehabilitation. While it briefly mentions some limitations of specific models, such as the difficulty of visualizing random forest results or interpreting support vector machines, a more thorough analysis of these challenges would provide a more balanced perspective.
The article also makes unsupported claims about the future use of machine learning in stroke rehabilitation. It suggests that machine learning will be actively used to predict depression occurrence and recovery of language, cognitive, and sensory function in stroke patients. However, there is no evidence provided to support these claims or discuss the feasibility and potential risks associated with using machine learning for these purposes.
Furthermore, the article does not adequately address potential risks or ethical considerations related to using machine learning in stroke rehabilitation. For example, it does not discuss issues related to data privacy and security when handling large amounts of clinical and imaging data. Additionally, there is no mention of potential biases or disparities that may arise from using machine learning algorithms trained on specific patient populations.
The article also lacks a balanced presentation of both sides of the argument regarding the use of machine learning in stroke rehabilitation. While it highlights some benefits and applications of machine learning, it does not thoroughly explore any potential drawbacks or criticisms associated with this approach. A more comprehensive analysis would have provided a more nuanced understanding of the topic.
In conclusion, while the article provides a useful overview of machine learning applications in stroke rehabilitation, it has several limitations and biases that should be critically analyzed. The limited focus on specific machine learning models, unsupported claims about future use, lack of discussion on limitations and challenges, and absence of a balanced presentation of both sides of the argument detract from the overall credibility and comprehensiveness of the article.