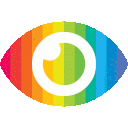
1. A natural language processing (NLP) algorithm can accurately identify silent brain infarcts (SBIs) and white matter disease (WMD) from neuroimaging reports.
2. Neuroimaging reports in routine clinical care contain reliable information on the presence and characteristics of SBIs and WMD.
3. The use of NLP algorithms can facilitate the identification of patients with SBIs and WMD for cohort development and research purposes.
The article titled "Agreement between neuroimages and reports for natural language processing-based detection of silent brain infarcts and white matter disease" discusses the use of natural language processing (NLP) algorithms to identify patients with silent brain infarcts (SBIs) and white matter disease (WMD) from neuroimaging reports. The authors aim to determine if these reports contain reliable information on these findings and if an NLP algorithm can accurately identify them.
One potential bias in this article is the selection of the study sample. The cohort consisted of patients older than 50 years with neuroimaging between 2009 and 2015 and no history of stroke, transient ischemic attack (TIA), or dementia. This specific selection criteria may limit the generalizability of the findings to a broader population.
Another potential bias is the reliance on radiology residents for report interpretation. While interrater reliability was assessed, it is unclear how experienced these residents were in interpreting neuroimaging reports. This could introduce variability in their interpretations and affect the accuracy of the NLP algorithm.
The article also lacks a discussion on potential limitations or sources of error in using NLP algorithms for identifying SBIs and WMD. NLP algorithms rely on accurate text recognition and understanding, which can be challenging when dealing with complex medical terminology. The accuracy of the algorithm may be affected by errors or inconsistencies in the text data it analyzes.
Additionally, there is limited discussion on the clinical implications of using NLP algorithms for cohort identification. While the article mentions that AI offers promising approaches for patient identification, it does not explore how this information can be used to improve patient care or inform treatment decisions.
Furthermore, there is no mention of any potential risks or ethical considerations associated with using AI algorithms in healthcare. It would have been valuable to discuss issues such as patient privacy, data security, and potential biases in algorithm development.
Overall, while this article provides some insights into the agreement between neuroimages and reports for the detection of SBIs and WMD using NLP algorithms, it has several limitations and biases that should be considered. Further research is needed to validate the findings in a larger and more diverse population and to address the potential limitations and risks associated with using AI algorithms in clinical practice.