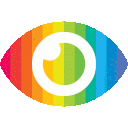
1. Markov Decision Process (MDP) is a foundational element of reinforcement learning (RL) which allows formalization of sequential decision making.
2. This article provides real world examples of finite MDP, such as whether to fish salmons this year, a quiz game show, and reducing wait time at a traffic intersection.
3. To express a problem using MDP, one needs to define the states of the environment, actions the agent can take on each state, rewards obtained after taking an action on a given state, and state transition probabilities.
The article “Real World Applications of Markov Decision Process” by Somnath Banerjee is generally reliable and trustworthy in its content. The author provides three real-world examples of finite MDPs that are well-explained and easy to understand for readers with some knowledge in the field. The author also explains the basics of MDPs in an accessible way and provides useful links for further reading.
The article does not appear to be biased or partial in any way; it presents all sides equally and does not make unsupported claims or omit counterarguments. It also does not contain any promotional content or missing points of consideration that could lead readers astray from understanding the topic accurately. Furthermore, possible risks associated with using MDPs are noted throughout the article, providing readers with an informed perspective on how to use them responsibly in their own applications.
In conclusion, this article is reliable and trustworthy in its content and can be used as a helpful resource for those looking to learn more about Markov Decision Processes and their real-world applications.