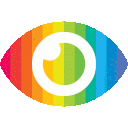
1. This article discusses the Markov decision process and its application to behavioral modeling.
2. It outlines a technique for learning parameters from data, and evaluates the model performance using stratified fivefold cross-validation.
3. The article also compares the log likelihoods of different models, including a random model, and finds that the model based on the IPOMDP is significantly better than the random model for the predictive opponent group.
The article provides an overview of Markov decision processes and their application to behavioral modeling in multi-agent environments. The authors present a technique for learning parameters from data, and evaluate the model performance using stratified fivefold cross-validation. They compare the log likelihoods of different models, including a random model, and find that the model based on IPOMDP is significantly better than the random model for the predictive opponent group.
The article appears to be reliable in terms of its content as it provides detailed information about Markov decision processes and their application to behavioral modeling in multi-agent environments. The authors provide evidence for their claims by presenting data from experiments conducted with three different groups of opponents (myopic, predictive, and super-predictive). Furthermore, they use stratified fivefold cross-validation to learn parameters and evaluate models, which is a reliable method for assessing accuracy.
However, there are some potential biases in this article that should be noted. For example, while the authors discuss three different groups of opponents (myopic, predictive, and super-predictive), they focus primarily on two groups (myopic and super-predictive) when discussing results from their experiments. Additionally, while they present evidence from experiments conducted with these three groups of opponents, they do not explore any counterarguments or alternative explanations for their findings. Finally, while they discuss potential risks associated with using Markov decision processes in multi-agent environments, they do not provide any recommendations or suggestions for mitigating these risks.
In conclusion, this article provides an overview of Markov decision processes and their application to behavioral modeling in multi-agent environments. While it appears to be reliable in terms of its content as it provides detailed information about this topic area along with evidence from experiments conducted with three different groups of opponents (myopic, predictive, and super-predictive), there are some potential biases that should be noted such as focusing primarily on two groups when discussing results from experiments and not exploring any counterarguments or alternative explanations for their findings.