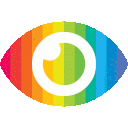
1. The use of mash-up language, such as Hinglish, on social media poses a challenge for automated text analytics and sentiment analysis.
2. Sarcasm detection is a specific type of sentiment analysis that requires detecting inconsistencies within opinion polarities.
3. The proposed softAttBiLSTM-feature-richCNN model combines bidirectional LSTM with soft attention mechanism and feature-rich CNN for automated sarcasm detection in Hinglish mash-up tweets.
The article titled "Sarcasm detection in mash-up language using soft-attention based bi-directional LSTM and feature-rich CNN" discusses the challenges of detecting sarcasm in social media posts, particularly in multilingual contexts. The article highlights the importance of sentiment analysis in social listening and the need for accurate detection of sarcasm, which is often expressed through non-standard vocabulary and informal textual content.
The article provides a comprehensive overview of the literature on sentiment analysis and sarcasm detection, highlighting the limitations of existing approaches in dealing with multilingual and code-mixed data. The authors propose a new model, softAttBiLSTM-feature-richCNN, which combines bidirectional long short-term memory with soft attention mechanism and feature-rich convolution neural network to detect sarcasm in Hinglish mash-up tweets.
While the proposed model appears promising, there are several potential biases and limitations that need to be considered. Firstly, the article focuses primarily on Hinglish mash-up tweets, which may not be representative of all multilingual contexts. Secondly, the authors rely heavily on pre-trained word embeddings such as GloVe without considering their potential biases or limitations. Thirdly, while the article acknowledges the challenges of detecting sarcasm accurately, it does not explore potential risks or ethical considerations associated with automated sentiment analysis.
Overall, while the article provides valuable insights into the challenges of detecting sarcasm in multilingual contexts and proposes a new model to address these challenges, it is important to consider its potential biases and limitations before applying it to real-world scenarios.