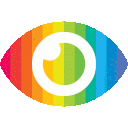
1. Sarcasm detection in low-resource languages like Hindi is a complex natural language processing task due to the scarcity of resources and tools.
2. A hybrid deep learning model trained using word and emoji embeddings can efficiently detect sarcasm in Hindi tweets with an accuracy of 97.35% and an F-score of 0.9708.
3. Emojis can provide useful contextual information for gauging sarcasm, analogous to human facial expressions, and automated feature engineering facilitates efficient and repeatable predictive models for detecting sarcasm in indigenous languages.
The article titled "Hybrid Deep Learning Model for Sarcasm Detection in Indian Indigenous Language Using Word-Emoji Embeddings" presents a study on the use of a hybrid deep learning model to detect sarcasm in Hindi tweets. The authors highlight the linguistic challenges of sarcasm detection in low-resource languages like Hindi, due to the scarcity of resources and tools such as annotated corpora, lexicons, dependency parser, Part-of-Speech tagger, and benchmark datasets. They propose using pre-trained word embeddings and emojis to capture contextual information for gauging sarcasm.
The article provides a detailed description of the methodology used in the study and reports promising results with an accuracy of 97.35% and an F-score of 0.9708. However, there are some potential biases and limitations that need to be considered.
Firstly, the study focuses only on Hindi tweets, which may not be representative of all forms of communication in the language. Additionally, the authors do not provide any information about how they selected their dataset or how they ensured its quality.
Secondly, while the use of emojis is an interesting approach to capturing contextual information for sarcasm detection, it is important to note that emojis can have different meanings depending on cultural context and interpretation. Therefore, it is unclear whether this approach would work equally well for other languages or cultures.
Thirdly, the article does not explore any potential ethical implications or risks associated with automated sarcasm detection. For example, there may be concerns around privacy violations or unintended consequences if such technology were used in real-world applications.
Overall, while the study presents an innovative approach to sarcasm detection in low-resource languages using deep learning models and emoji embeddings, it is important to consider its potential biases and limitations before drawing any definitive conclusions about its effectiveness or generalizability.