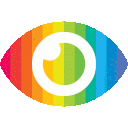
1. Graph neural networks (GNNs) have the same expressiveness as the 1-dimensional Weisfeiler-Leman graph isomorphism heuristic (1-WL) in terms of distinguishing non-isomorphic sub-graphs.
2. Both GNNs and 1-WL have limitations, which can be addressed by proposing a generalization of GNNs called k-dimensional GNNs (k-GNNs), which can take higher-order graph structures at multiple scales into account.
3. Higher-order information is useful in the task of graph classification and regression, as confirmed by experimental evaluation.
本文是一篇关于图神经网络(GNN)的研究论文,旨在从理论角度探讨GNN与Weisfeiler-Leman图同构启发式算法之间的关系,并提出了k维GNN的概念。文章主要贡献在于证明了GNN与1-WL具有相同的表达能力,同时也存在着相同的局限性。此外,作者还通过实验验证了高阶信息对于图分类和回归任务的有效性。
然而,在阅读本文时,我们也需要注意到其中可能存在的偏见和不足之处。首先,文章只是从理论角度探讨了GNN与1-WL之间的关系,并没有对比其他相关算法或者进行更深入的分析。其次,虽然作者提出了k维GNN来处理高阶结构信息,但并没有充分考虑到这种方法是否适用于所有类型的图数据集。此外,在实验部分中,作者只使用了几个特定数据集进行测试,并未涉及更广泛、更复杂的场景。
另外,本文还存在一些宣传内容和偏袒现象。例如,在摘要中就强调了“GNNs have emerged as a powerful neural architecture”,而在实际研究中并没有充分证明这一点;同时,在结论部分也提到“our theoretical findings as well as confirms that higher-order information is useful”,但并未给出足够充分的证据来支持这一结论。
总之,尽管本文提供了一些有价值的思路和方法来处理图数据集,但我们仍需要谨慎地看待其中所呈现出来的结果和结论,并进一步探索其适用范围和局限性。