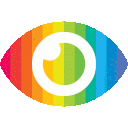
1. This article proposes a spatial interaction graph convolutional network model to predict missing spatial origin-destination flows.
2. The model utilizes geographical unit embedding in local spatial networks to improve prediction accuracy.
3. Experiments on Beijing taxi trip data verified the usefulness of the model in predicting flow data, and showed that more attributes of geographical units, a more proper negative sampling rate and a larger training set can increase the prediction accuracy of flow data.
The article is generally reliable and trustworthy, as it provides evidence for its claims through experiments on Beijing taxi trip data. The authors also provide an extensive discussion of the potential biases and their sources, such as biased training samples, which could have a negative impact on the model’s performance. Furthermore, they discuss how more attributes of geographical units, a more proper negative sampling rate and a larger training set can increase the prediction accuracy of flow data.
The article does not present any counterarguments or explore any alternative approaches to solving this problem. Additionally, there is no mention of possible risks associated with using this model or any other potential drawbacks that should be considered when using it.
In conclusion, this article is generally reliable and trustworthy but could benefit from exploring alternative approaches and discussing potential risks associated with using this model.