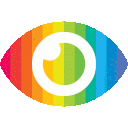
1. Precise noise estimation in Kalman Filter (KF) is not always equivalent to minimizing filtering errors, as even small violations of KF assumptions can significantly modify the effective noise.
2. Gradient-based optimization directly applied to filtering errors with an efficient parameterization of KF's symmetric and positive-definite parameters can improve accuracy and robustness in state-estimation and tracking problems.
3. Optimized KF can outperform complicated models like neural networks, which may seem superior but are merely more optimized.
作为一篇科技论文,该文章的内容相对客观,但仍存在一些潜在偏见和缺失的考虑点。
首先,文章强调了噪声估计的不足之处,并提出了优化KF参数的方法。然而,文章并没有充分探讨噪声估计方法的优缺点,也没有提供足够的证据来支持其主张。此外,文章未考虑到优化KF参数可能会带来新的风险和挑战。
其次,文章将KF与神经网络模型进行比较,并得出结论称优化KF可以消除神经网络模型看似更好的表现。然而,这种比较可能存在片面性和不公平性。神经网络模型和KF有着不同的应用场景和优劣势,在某些情况下神经网络模型确实可以更好地解决问题。
最后,文章未探讨KF在实际应用中可能遇到的复杂性和挑战。例如,在处理非线性系统或具有非高斯噪声时,KF可能无法很好地工作。因此,在实际应用中需要综合考虑多种因素来选择合适的方法。
总之,尽管该文章提供了一种新颖且有趣的思路来解决KF中噪声估计问题,但仍需要更全面、客观地评估其优缺点,并注意到可能存在的风险和挑战。