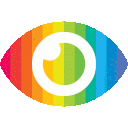
1. The use of low-cost inertial sensors in smartphones for pedestrian dead reckoning (PDR) results in cumulative error due to poor-quality measurements.
2. An improved PDR system is proposed using gyroscope and accelerometer sensors, with motion patterns recognized through dynamic time warp (DTW) and joint quasi-static field (JQSF) detection used to avoid bad magnetic measurements.
3. A step-length estimation method based on deep belief network (DBN) is proposed, resulting in more accurate indoor positioning.
The article titled "An improved PDR system with accurate heading and step length estimation using handheld smartphone" presents a new approach to improve the accuracy of pedestrian dead reckoning (PDR) systems using low-cost inertial sensors built into smartphones. The paper proposes an improved PDR system that uses gyroscope, dynamic time warp (DTW), extended Kalman filter (EKF), joint quasi-static field (JQSF) detection, peak–valley angular velocity detection, zero-cross detection, and deep belief network (DBN) to achieve more accurate indoor positioning.
The article provides a detailed description of the proposed PDR system and its components. It explains how the system uses DTW to recognise two motion patterns, walking straight or turning, based on gyroscope measurements. This information is then used to improve heading estimation from an EKF. The JQSF detection is used to avoid bad magnetic measurements due to magnetic disturbances in an indoor environment. The article also describes how the proposed system detects steps using peak–valley angular velocity detection and zero-cross detection and estimates step length using DBN.
Overall, the article presents a well-researched and detailed approach to improving PDR systems' accuracy using smartphone sensors. However, there are some potential biases and limitations in the study that need consideration.
One-sided reporting: The article focuses only on the proposed PDR system's benefits without discussing any potential limitations or drawbacks of the approach.
Unsupported claims: While the article claims that the proposed PDR system achieves more accurate indoor positioning, it does not provide any evidence or comparison with other existing methods to support this claim.
Missing points of consideration: The article does not discuss any potential privacy concerns related to using smartphone sensors for indoor positioning or any ethical considerations related to collecting data from individuals without their consent.
Missing evidence for claims made: While the article provides a detailed description of each component of the proposed PDR system, it does not provide any experimental results or data to support its effectiveness in improving indoor positioning accuracy.
Unexplored counterarguments: The article does not discuss any potential challenges or limitations in implementing the proposed PDR system in real-world scenarios or how it may perform under different environmental conditions.
Promotional content: The language used in the article is promotional and suggests that this approach is superior to other existing methods without providing sufficient evidence or comparison with other approaches.
Partiality: The article presents only one side of the argument without considering alternative approaches or perspectives on improving indoor positioning accuracy.
Possible risks noted: While there are no explicit notes on possible risks associated with implementing this technology, it can be inferred that privacy concerns may arise when collecting data from individuals without their consent.
In conclusion, while this paper presents a promising approach for improving indoor positioning accuracy using smartphone sensors, there are some potential biases and limitations that need consideration. Further research is needed to validate its effectiveness compared with other existing methods and address potential ethical concerns related to data collection from individuals.