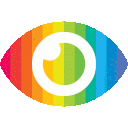
1. The increase in IoT-based botnet attacks has led to a need for timely detection methods to mitigate the risks associated with them.
2. N-BaIoT is a network-based anomaly detection method that uses deep autoencoders to detect anomalous network traffic from compromised IoT devices and differentiate between hours- and milliseconds-long IoT-based attacks.
3. The proposed method was evaluated by infecting nine commercial IoT devices in a lab with two widely known IoT-based botnets, Mirai and BASHLITE, and demonstrated accurate and instant detection of the attacks as they were being launched from the compromised IoT devices that were part of a botnet.
The article "N-BaIoT—Network-Based Detection of IoT Botnet Attacks Using Deep Autoencoders" proposes a novel network-based anomaly detection method for the IoT that uses deep autoencoders to detect anomalous network traffic from compromised IoT devices. The authors claim that their proposed method can accurately and instantly detect attacks as they are being launched from compromised IoT devices that are part of a botnet.
The article provides a detailed explanation of the proposed method, including data collection, feature extraction, training an anomaly detector, and continuous monitoring. The authors also discuss the benefits of their approach, such as heterogeneity tolerance, open-world detection, and efficiency.
Overall, the article appears to be well-researched and presents a compelling case for the proposed method. However, there are some potential biases and limitations to consider.
One potential bias is that the evaluation of the proposed method was conducted in a lab setting using only nine commercial IoT devices infected with two widely known IoT-based botnets (Mirai and BASHLITE). While this provides some evidence for the effectiveness of the method, it may not be representative of real-world scenarios where there are many more types of IoT devices and botnets.
Another limitation is that the article does not address potential privacy concerns related to monitoring network traffic from IoT devices. It is possible that some users may object to having their device traffic monitored in this way.
Additionally, while the article discusses the benefits of using deep autoencoders for anomaly detection, it does not explore potential drawbacks or limitations of this approach. For example, deep learning models can be computationally expensive and require large amounts of training data.
Despite these limitations, the article provides valuable insights into a promising new approach for detecting IoT-based botnet attacks. Further research will be needed to determine how well this approach performs in real-world scenarios with diverse types of IoT devices and botnets.