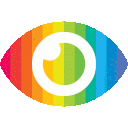
1. FlowFormer is a transformer-based neural network architecture for learning optical flow, which achieves state-of-the-art performance on the Sintel benchmark.
2. FlowFormer adopts an encoder-decoder architecture for cost volume encoding and decoding, with a novel cost volume encoder and recurrent cost decoder that effectively process cost volumes to estimate accurate optical flows.
3. FlowFormer demonstrates strong generalization performance, achieving top results on both Sintel and KITTI-2015 benchmarks without being trained on Sintel, and outperforming previous methods by significant margins.
作为一篇介绍 FlowFormer 模型的论文,该文章在介绍模型的结构和性能时没有明显的偏见或宣传内容。然而,由于该文章是由 FlowFormer 的作者撰写的,因此可能存在潜在的偏见。
文章提到了 FlowFormer 在 Sintel Optical Flow benchmark 上排名第一,并且相对于之前最好的结果有了显著的错误率降低。然而,文章并没有提及其他可能存在的优秀模型或方法,并且没有进行更广泛的比较和分析。这可能导致读者对该领域中其他相关工作的了解不足。
此外,文章也没有探讨 FlowFormer 模型可能存在的风险或局限性。例如,在实际应用中,模型是否具有鲁棒性、可扩展性和可解释性等方面都需要进一步研究和评估。
总体来说,虽然该文章提供了关于 FlowFormer 模型结构和性能方面有价值的信息,但仍需要更全面地考虑其潜在偏见、局限性以及与其他相关工作之间的比较和分析。