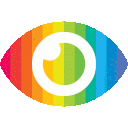
1. A lightweight end-to-end pest detection algorithm, YOLOv4_MF, is proposed based on the You Only Look Once (YOLO) algorithm to improve the efficiency of pest detection.
2. MobileNetv2 embedded with coordinate attention was used as the feature extraction network to increase the mobile detection speed of a deployed model.
3. The BA block was designed as the multi-scale feature fusion network to integrate semantic and detailed information of small targets and introduce as few parameters as possible.
The article presents a new lightweight YOLOv4-based forestry pest detection method using coordinate attention and feature fusion. The authors provide a comprehensive review of traditional and deep learning-based methods for pest detection, highlighting the limitations of existing approaches. They propose a new algorithm that addresses the challenges of detecting small targets, balancing recognition effectiveness and light weight, and uneven distribution of positive and negative samples in the dataset.
The article is well-written and provides detailed explanations of the proposed approach, including the backbone network (MobileNetv2), attention mechanism (coordinate attention), feature fusion (BA block), and loss function (focal loss). The authors also compare their approach with YOLOv4 on a pest dataset, demonstrating improved performance in terms of mean average precision (mAP) and frame per second (FPS).
However, there are some potential biases in the article that need to be considered. Firstly, the authors only focus on visual image-based methods for pest detection, ignoring other sensor-based methods that may have advantages in certain scenarios. Secondly, while they acknowledge the challenges of detecting small targets, they do not explore potential counterarguments or alternative solutions to this problem. Thirdly, they do not discuss any possible risks or limitations associated with their approach.
Overall, while the article presents an interesting new approach to forestry pest detection using deep learning techniques, it is important to consider its potential biases and limitations when evaluating its applicability in real-world scenarios.