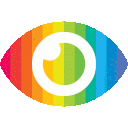
1. This study uses a long short-term memory (LSTM) neural network architecture to predict the next-day closing price of the S&P 500 index.
2. A well-balanced combination of nine predictors is carefully constructed under the umbrella of fundamental market data, macroeconomic data, and technical indicators.
3. The experimental results show that the single layer LSTM model provides a superior fit and high prediction accuracy compared to multilayer LSTM models.
The article “Predicting stock market index using LSTM” is an interesting exploration into how machine learning techniques can be used to predict stock prices. The article presents a comprehensive overview of the research conducted on this topic, including a detailed description of the methodology used and its results. However, there are some potential biases in the article that should be noted.
First, it is important to note that the article does not present both sides equally when discussing stock market predictions. While it does mention some potential risks associated with predicting stock prices, such as collinearity among multiple variables, it does not explore any counterarguments or alternative approaches to predicting stock prices. This could lead readers to believe that this approach is the only viable option for predicting stock prices when in fact there may be other methods available that could yield better results.
Second, while the article does provide evidence for its claims regarding the performance of single layer and multilayer LSTM models, it does not provide any evidence for its claims about how certain variables correlate with stock price behavior or why certain explanatory variables were chosen over others. This lack of evidence could lead readers to question whether these claims are supported by actual data or if they are simply assumptions made by the authors without any supporting evidence.
Finally, it is also important to note that while this article provides an interesting exploration into how machine learning techniques can be used to predict stock prices, it does not discuss any potential ethical implications associated with using such techniques in financial markets or address any possible risks associated with relying too heavily on automated predictions rather than human judgement when making investment decisions. These points should have been addressed in order for readers to gain a more complete understanding of this topic and its implications for financial markets as a whole.