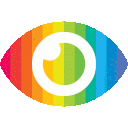
1. The gasification process for biomass involves multiple simultaneous reactions and complex variables, making direct simulation computationally expensive.
2. Surrogate models generated using regression techniques, such as Gaussian process regression, artificial neural networks, and random forest regression, can accurately predict the behavior of biomass gasification systems.
3. The surrogate models demonstrated lower mean squared error and uncertainty compared to direct simulation, making them a more efficient and cost-effective solution for optimizing biomass gasification processes.
The article titled "Comparison of regression techniques for generating surrogate models to predict the thermodynamic behavior of biomass gasification systems" discusses the use of surrogate models in predicting the behavior of biomass gasification systems. The article provides an overview of the complexities involved in modeling gasification processes and highlights the benefits of using surrogate models to accelerate optimization processes.
One potential bias in this article is the lack of discussion on the limitations and uncertainties associated with surrogate models. While the article mentions that uncertainty is quantified in the surrogate models, it does not provide a thorough analysis of how these uncertainties can impact the accuracy and reliability of the predictions. This omission could lead readers to believe that surrogate models are a foolproof solution, when in reality they have their own limitations.
Another potential bias is the focus on only three regression techniques (Gaussian process regression, Artificial neural networks, and Random Forest regression) for generating surrogate models. There are numerous other regression techniques available, and it would be beneficial to explore their applicability and performance in this context. By limiting the discussion to only three techniques, the article may be presenting a biased view of the effectiveness of surrogate models.
Additionally, there is a lack of discussion on potential risks or drawbacks associated with using surrogate models in industrial systems. Surrogate models rely on simplifications and assumptions, which may not always capture all aspects of complex processes accurately. It would be important to address these risks and provide a balanced view of both the advantages and disadvantages of using surrogate models.
Furthermore, there is limited discussion on alternative approaches or methods for predicting the thermodynamic behavior of biomass gasification systems. The article focuses solely on surrogate models without considering other modeling techniques or approaches that may also be applicable in this context. This narrow focus limits the scope of the analysis and prevents a comprehensive evaluation of different methods.
Overall, while this article provides some insights into using surrogate models for predicting biomass gasification systems' behavior, it has several biases and limitations. The lack of discussion on the limitations and uncertainties of surrogate models, the limited focus on only three regression techniques, the absence of a comprehensive analysis of alternative approaches, and the omission of potential risks associated with surrogate models all contribute to a one-sided and incomplete view of the topic.