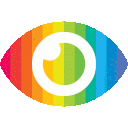
1. The Stable Diffusion 2.0 release includes improved text-to-image models with higher quality generated images.
2. The release also features an Upscaler Diffusion model that enhances image resolution by a factor of 4.
3. The depth-guided stable diffusion model, called depth2img, allows for creative applications by generating images using both text and depth information.
The article titled "Stable Diffusion 2.0 Release — Stability AI" introduces the new features and improvements in the Stable Diffusion 2.0 release by Stability AI. While the article provides information about the new text-to-image models, super-resolution upscaler diffusion models, depth-to-image diffusion model, and updated inpainting diffusion model, it is important to critically analyze the content for potential biases, unsupported claims, missing evidence, and promotional content.
One potential bias in the article is the lack of information about any limitations or drawbacks of the Stable Diffusion 2.0 release. The article focuses solely on highlighting the new features and improvements without mentioning any potential risks or challenges associated with using these models. This one-sided reporting can create an overly positive impression of the product while ignoring its limitations.
Additionally, there are several unsupported claims in the article. For example, it states that the text-to-image models in this release greatly improve image quality compared to earlier versions without providing any evidence or comparative analysis to support this claim. Similarly, it claims that combining text-to-image models with upscaler diffusion models can generate images with resolutions of 2048x2048 or higher but does not provide any examples or evidence to demonstrate this capability.
The article also lacks exploration of counterarguments or alternative perspectives. It presents Stable Diffusion 2.0 as a powerful tool for creative applications without discussing any potential concerns or criticisms that may exist regarding generative AI models. This omission limits a comprehensive understanding of both sides of the argument and can be seen as partial reporting.
Furthermore, there is a promotional tone throughout the article, emphasizing how amazing things can be created when millions of people have access to these models and highlighting Stability AI's commitment to open source development. While promoting their work is understandable from a marketing perspective, it is important to critically evaluate whether certain claims are supported by evidence or if they are merely promotional statements.
The article also lacks specific evidence or examples to support some of the claims made. For instance, it mentions that the text-to-image models are trained on an aesthetic subset of the LAION-5B dataset and filtered to remove adult content but does not provide any details or evidence regarding the effectiveness of this filtering process.
Overall, the article presents an overview of the new features and improvements in Stable Diffusion 2.0 but lacks critical analysis, balanced reporting, and supporting evidence for some of its claims. It is important for readers to approach the information with a critical mindset and seek additional sources or evidence before forming conclusions about the capabilities and limitations of Stable Diffusion 2.0.