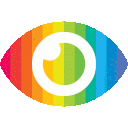
1. IoT architecture: The article introduces a three-layer IoT architecture consisting of the thing layer, fog layer, and cloud layer, which enables data collection and processing from various IoT devices with limited resources.
2. Machine learning for IoT security: The article discusses the use of machine learning algorithms for detecting IoT attacks, highlighting the limitations of traditional ML techniques in real-time predictions and low-resource environments.
3. Ensemble model for IoT attack detection: The article proposes an ensemble model for detecting IoT attacks that does not require additional feature extraction or dimensional reduction algorithms and is best suited for real-time applications with limited resources. Extensive experiments on the NSL-KDD dataset show high accuracy in minimum time with fewest possible resources needed.
该文章提出了一个针对物联网攻击的集成机器学习模型,旨在实现快速检测和准确性。然而,该文章存在一些潜在的偏见和缺陷。
首先,该文章没有充分考虑到物联网系统中可能存在的隐私和安全风险。尽管作者提到了物联网安全解决方案,但并未深入探讨这些解决方案是否足够保护用户数据和隐私。此外,该文章也没有探讨可能的攻击类型或攻击者的动机。
其次,该文章只使用了NSL-KDD数据集进行实验,并未考虑其他可能存在的数据集或真实场景下的情况。这种局限性可能导致结果不够全面或适用于所有情况。
此外,该文章没有提供足够的证据来支持其主张。例如,在介绍深度学习算法时,作者声称它们比传统机器学习算法更有效地检测物联网攻击,但并未提供任何具体证据来支持这一点。
最后,该文章似乎偏袒使用集成机器学习模型来检测物联网攻击,并未探讨其他可能的解决方案或方法。这种偏袒可能导致读者对该模型的效果和适用性产生过高的期望。
综上所述,该文章存在一些潜在的偏见和缺陷,需要更全面地考虑物联网系统中可能存在的风险和挑战,并提供更充分的证据来支持其主张。