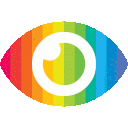
1. DreamBooth is a personalized text-to-image model that can synthesize photorealistic images of a subject in different contexts by fine-tuning a pretrained model with just a few input images and a class name.
2. The approach leverages the semantic prior embedded in the model with a class-specific prior preservation loss to enable synthesizing diverse instances of the subject while preserving its key features.
3. DreamBooth can be applied to various tasks, including subject recontextualization, text-guided view synthesis, appearance modification, and artistic rendering, while preserving the subject's identity and essence. However, there are concerns about potential misuse of such technology for malicious purposes.
The article "DreamBooth: Fine Tuning Text-to-image Diffusion Models for Subject-Driven Generation" presents a new approach for personalizing text-to-image diffusion models to generate photorealistic images of subjects in different contexts. The authors claim that their method can synthesize diverse instances of a subject, modify its appearance, and create artistic renditions while preserving the subject's key features.
The article provides a clear background on the limitations of current text-to-image models in generating diverse instances of a given subject. However, it is unclear whether the authors have considered all existing approaches to address this limitation. Additionally, the article lacks evidence to support their claim that their method outperforms other existing methods.
The approach presented in the article involves fine-tuning a pretrained text-to-image model with just a few images of a subject and its corresponding class name. The authors claim that their technique enables synthesizing the subject in diverse scenes, poses, views, and lighting conditions that do not appear in the reference images. However, there is no clear explanation or evidence provided on how this is achieved.
The results presented in the article show impressive outputs generated by their method for various tasks such as re-contextualization, artistic rendering, property modification, accessorization, and text-guided view synthesis. However, it is unclear whether these results are representative of all possible scenarios or if they are cherry-picked examples.
The societal impact section acknowledges potential risks associated with using generative models to mislead viewers but does not provide any concrete steps or recommendations to mitigate these risks.
Overall, while the article presents an interesting approach for personalizing text-to-image diffusion models and provides impressive results for various tasks, it lacks sufficient evidence and consideration of existing approaches to support its claims fully. Additionally, more attention could be given to potential risks associated with using generative models for malicious purposes.