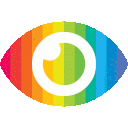
1. The article proposes an automated dermoscopic Skin Lesion Classification (SLC) framework named DermoExpert that combines pre-processing and a hybrid Convolutional Neural Network (hybrid-CNN).
2. The proposed pre-processing includes lesion segmentation, augmentation, and class rebalancing, while the hybrid-CNN employs three distinct feature extractor modules fused to achieve better-depth feature maps of the lesion.
3. DermoExpert outperforms state-of-the-art results on ISIC-16 (2-class), ISIC-17 (3-class), and ISIC-18 (7-class) datasets, providing a better recognition tool to assist dermatologists.
The article titled "DermoExpert: Skin lesion classification using a hybrid convolutional neural network through segmentation, transfer learning, and augmentation" presents a new framework for automated skin lesion classification (SLC) using dermoscopic images. The proposed framework combines pre-processing techniques such as lesion segmentation, augmentation, and class rebalancing with a hybrid Convolutional Neural Network (CNN) that employs transfer learning and ensembling to achieve state-of-the-art results on three different datasets.
The article provides a comprehensive review of related works in the field of SLC-CAD systems and highlights the limitations of existing methods. The authors argue that handcrafted feature-based methods require extensive feature engineering, which is challenging due to the appearance of various artifacts in dermoscopic images. On the other hand, CNN-based methods suffer from data scarcity limitations to avoid overfitting. The authors propose an end-to-end ensemble strategy without compromising state-of-the-art results to mitigate these weaknesses.
The article's strengths lie in its detailed description of the proposed framework's components and their contributions to achieving better SLC results. The authors provide experimental results that demonstrate the effectiveness of their approach compared to existing methods. They also make their source code and segmented masks for the ISIC-2018 dataset available as a public benchmark for future improvements.
However, there are some potential biases in the article that need consideration. Firstly, the authors do not discuss any possible risks associated with automated SLC systems or address any ethical concerns related to privacy or data protection. Secondly, while they acknowledge that CNN-based methods suffer from data scarcity limitations, they do not explore alternative approaches such as generative adversarial networks (GANs) or semi-supervised learning that can leverage unlabeled data to improve performance.
In conclusion, the article presents a promising new framework for automated SLC using dermoscopic images that achieves state-of-the-art results on multiple datasets. However, it is essential to consider potential biases and limitations in the proposed approach and explore alternative methods to address them.