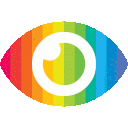
1. Spam emails pose a serious threat to users as they can lead to theft of user credentials and other malicious attacks.
2. A Natural Language Processing based Random Forest approach can be used to detect spam emails and temporary email addresses.
3. This approach improves the accuracy of spam email filtering by detecting natural languages spoken by people and using multiple decision trees for filtering spams.
The article titled "Detection of Email Spam using Natural Language Processing Based Random Forest Approach" discusses the problem of spam emails and proposes a novel approach for detecting and filtering them. While the topic is relevant and important, there are several issues with the article that need to be addressed.
Firstly, the article lacks proper citations and references. It mentions that certain methods and techniques are used for spam detection, but does not provide any sources or evidence to support these claims. This raises questions about the credibility of the information presented.
Secondly, the article makes unsupported claims about the effectiveness of the proposed approach. It states that the use of Natural Language Processing (NLP) and Random Forest can improve the accuracy of spam email filtering, but does not provide any data or experiments to support this claim. Without empirical evidence, it is difficult to assess the validity of these claims.
Additionally, the article does not explore potential counterarguments or limitations of the proposed approach. It presents NLP-RF as a solution without discussing any potential drawbacks or challenges that may arise in its implementation. This one-sided reporting undermines the credibility of the article.
Furthermore, there is a lack of discussion on potential risks or ethical considerations associated with spam detection methods. For example, false positives can result in legitimate emails being classified as spam, leading to important messages being missed by users. The article does not address these concerns or provide any insights into how they can be mitigated.
Moreover, there is a promotional tone throughout the article, suggesting that NLP-RF is an efficient and effective solution for spam detection without providing sufficient evidence to support this claim. This partiality undermines the objectivity of the article.
In conclusion, while the topic of spam email detection is important, this particular article lacks proper citations, provides unsupported claims, ignores potential counterarguments and risks, and has a promotional tone. These issues undermine its credibility and make it difficult to fully assess its content.