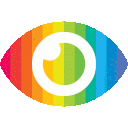
1. The PathFinder framework, a deep learning model, has been developed to assess prognosis and discover biomarkers for liver cancer.
2. PathFinder uses the macro mode of whole slide images (WSIs) to achieve high-precision prognosis and identifies spatial distribution of necrosis as a significant indicator for clinical prognosis.
3. The framework combines multi-class tissue spatial distribution information with attribution maps to provide interpretable results and guide the discovery of novel biomarkers for liver cancer prognosis.
The article titled "Deep learning supported discovery of biomarkers for clinical prognosis of liver cancer" discusses the development of an interpretable deep learning framework called PathFinder for identifying biomarkers in liver cancer prognosis. While the article provides valuable insights into the potential of artificial intelligence (AI) in improving clinical prognosis, there are several aspects that need critical analysis.
One potential bias in the article is its focus on promoting the use of AI-based algorithms in clinical practice without adequately addressing their limitations. The authors emphasize the need to augment human intelligence rather than replace it, but they do not thoroughly discuss the challenges and risks associated with relying solely on AI algorithms for diagnosis and prognosis. This one-sided reporting may create unrealistic expectations about the capabilities and reliability of AI systems.
Furthermore, the article claims that current tissue biomarkers for liver cancer prognosis lack robustness, sensitivity, and specificity. However, it does not provide sufficient evidence or references to support this claim. Without proper validation and comparison with existing biomarkers, it is difficult to assess whether the proposed AI-based approach truly outperforms current methods.
The article also lacks a comprehensive discussion on potential ethical considerations and risks associated with using AI algorithms in healthcare. For example, there is no mention of issues such as data privacy, algorithmic bias, or potential harm caused by incorrect predictions. These considerations are crucial when implementing AI systems in real-world clinical settings.
Additionally, while the article highlights the importance of interpretability in AI models, it does not delve into how PathFinder achieves this interpretability or provide concrete examples of how clinicians can use this information to make informed decisions. Without a detailed explanation of the interpretability mechanisms employed by PathFinder and their practical implications, it is challenging to evaluate its effectiveness as a tool for biomarker discovery.
Moreover, there is limited discussion on potential counterarguments or alternative approaches to biomarker discovery in liver cancer prognosis. The article presents PathFinder as a novel solution without exploring other existing methods or considering their comparative advantages and disadvantages. This lack of comprehensive analysis limits the reader's understanding of the broader landscape of biomarker discovery in cancer prognosis.
In conclusion, while the article introduces an interesting AI-based framework for biomarker discovery in liver cancer prognosis, it has several limitations and biases that need to be critically analyzed. The article's focus on promoting AI without adequately addressing its limitations, lack of evidence for claims made, insufficient discussion on ethical considerations and risks, and limited exploration of alternative approaches all contribute to a one-sided and potentially biased presentation of the topic.