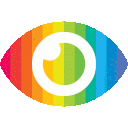
1. The article proposes a method for early detection and localization of Inter-Turn Short Circuit (ITSC) faults in the stator winding of induction motors.
2. The method uses a novel indicator based on the Discrete Wavelet Energy Ratio (DWER) of three stator currents, with Artificial Neural Network (ANN).
3. Experimental tests were carried out to confirm the effectiveness of the proposed method, which proved to be accurate and robust in detecting and localizing ITSC faults even under different operating conditions of the motor.
The article proposes a new method for early detection and localization of inter-turn short circuit (ITSC) faults in the stator winding of induction motors. The proposed method uses a novel indicator based on the Discrete Wavelet Energy Ratio (DWER) of three stator currents, with Artificial Neural Network (ANN). The authors claim that their method is simple, low-cost, and accurate in detecting even a low number of shorted turns faults in the stator winding of the motor.
The article provides a comprehensive review of related literature and explains the advantages and disadvantages of different fault detection techniques. The authors justify their choice of using ANN as an alternative approach to represent knowledge about faults due to its ability to filter disturbances and noise without complicated mathematical models.
The experimental setup and tests are described in detail, which adds credibility to the study. The results obtained from the experiments show that DWER is an accurate and robust indicator to diagnose ITSC faults. The authors also compare their proposed ENN model with traditional feedforward neural networks MLP and RBF neural networks, confirming its efficiency in diagnosing ITSC faults.
However, there are some limitations to this study. Firstly, the sample size used for testing is relatively small, which may not be representative enough to generalize the findings. Secondly, there is no discussion on potential risks associated with using ANN for fault diagnosis or any ethical considerations related to this technology.
Moreover, while the article presents a detailed explanation of how their proposed method works, it does not explore any counterarguments or limitations that may arise when implementing this technique in real-world scenarios. Additionally, there is no discussion on how this method can be integrated into existing predictive maintenance strategies or how it compares with other methods currently used by industry professionals.
In conclusion, while the proposed method shows promise in detecting ITSC faults accurately and efficiently, further research is needed to validate its effectiveness on larger samples sizes and under different operating conditions. Additionally, the authors should consider discussing potential risks and ethical considerations associated with using ANN for fault diagnosis, as well as exploring any limitations or counterarguments that may arise when implementing this technique in real-world scenarios.