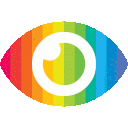
1. Self-attention mechanism has been a key factor in the recent progress of Vision Transformer (ViT), but existing self-attention methods may compromise local feature learning or subject to some handcrafted designs.
2. Local attention, which restricts the receptive field of each query to its own neighboring pixels, enjoys the benefits of both convolution and self-attention, but current local attention modules either use inefficient Im2Col function or rely on specific CUDA kernels that are hard to generalize to devices without CUDA support.
3. The proposed Slide Attention module leverages common convolution operations to achieve high efficiency, flexibility and generalizability for local attention, and achieves consistently improved performances on comprehensive benchmarks for various advanced Vision Transformer models and hardware devices.
作为一篇技术论文,本文主要介绍了一种新的局部注意力模块Slide Attention,并探讨了其在视觉Transformer模型中的应用。文章提出了一个新的思路,即从行的角度重新解释Im2Col函数,使用深度卷积代替移位操作,从而实现高效、灵活和通用的局部注意力机制。作者通过大量实验证明了该方法在各种视觉任务中都具有优异的性能表现。
然而,在阅读本文时需要注意到以下几点:
首先,本文是一篇技术论文,主要关注于算法和技术方面,对于社会、伦理等方面并没有涉及。因此,在评价本文时需要将重点放在其技术贡献上。
其次,虽然作者提出了一种新的局部注意力模块Slide Attention,并证明其在各种视觉任务中都具有优异的性能表现,但是文章并没有与其他已有的局部注意力模块进行充分比较和分析。因此,在评价该方法时需要考虑到可能存在其他更好或更适合特定任务的局部注意力模块。
另外,在介绍全局注意力和窗口注意力时,作者提到它们存在一些缺陷。然而,在实际应用中这些方法也具有很高的效率和性能表现,因此需要在评价局部注意力模块时进行客观比较和权衡。
最后,本文并没有探讨该方法可能存在的风险或局限性,也没有平等地呈现双方观点。因此,在阅读本文时需要保持批判性思维,不仅要看到其技术贡献,还要考虑到可能存在的问题和争议。