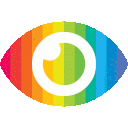
1. The article discusses emergent behavior in various contexts, including tumor growth and machine learning systems.
2. In the context of tumor growth, different hallmarks can lead to different emergent behaviors, such as evasion of apoptosis or effective immortality.
3. Emergent behavior in machine learning systems can be affected by interagent dependencies and biases, and may require a holistic approach to system design.
The article provides an overview of emergent behavior in various contexts, including tumor growth, machine learning, artificial intelligence, and decision analysis. The section on tumor growth uses simulations to analyze the emergence of different cancer hallmarks and their impact on tumor progression. However, the article does not provide a comprehensive analysis of all possible scenarios and only focuses on three representative situations.
The section on machine learning discusses the potential for emergent behavior in neural network-based agents and the importance of understanding interagent dependencies for accurate ML/NN estimators. However, the article does not explore counterarguments or potential risks associated with using AI and ML systems.
The section on artificial intelligence presents two major groups of approaches to AI: symbol-processing approaches and subsymbolic approaches. The article briefly discusses their differences but does not provide a comprehensive analysis of their strengths and weaknesses.
The section on decision analysis describes an experiment with a robot that exhibits emergent behavior in response to its environment. However, the article does not provide sufficient evidence or details about the experiment to fully understand its implications.
Overall, while the article provides some interesting insights into emergent behavior in various contexts, it lacks depth and thoroughness in its analysis. It also appears to have a bias towards certain approaches or technologies without fully exploring alternative perspectives or potential risks.