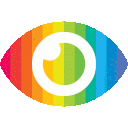
1. Alzheimer's disease is a prevalent neurodegenerative disease that impairs memory, thinking, and language, and efficient, noninvasive, and scalable practices for its diagnosis are essential.
2. Machine learning is being employed in the medical domain to create a supplementary tool for disease diagnosis, but collecting and labeling medical data is costly and labor-intensive. Transfer learning can effectively transfer information between domains considering data distribution discrepancy to build predictive models in a target dataset with a limited amount of data.
3. Transfer learning approaches can be classified into instance-based, feature-based, or model-based techniques. Deep learning models such as convolutional neural networks (CNNs) have been extensively used for the automatic extraction of discriminative features and classification, making them ideal candidates for transfer learning.
本文介绍了一种基于梯度提升机的迁移学习方法,用于阿尔茨海默病的诊断。文章首先介绍了阿尔茨海默病的背景和诊断的重要性,然后讨论了机器学习在医学领域中的应用以及迁移学习在处理医学数据中遇到的挑战。接着,文章详细介绍了不同类型的迁移学习方法,并提到了一些相关研究。最后,文章介绍了作者们提出的基于梯度提升机的迁移学习方法,并给出了实验结果。
总体来说,这篇文章对阿尔茨海默病诊断和机器学习在医学领域中的应用进行了较为全面和深入地探讨。然而,在某些方面存在一些偏见或缺失考虑点:
1. 偏见来源:文章没有明确提到阿尔茨海默病可能与生活方式、环境等因素有关,而过分强调其与年龄有关。这可能会导致读者忽略其他可能影响该疾病发生和发展的因素。
2. 片面报道:文章只涉及到神经影像方面的数据处理和分析方法,没有考虑其他类型数据(如基因组数据)对阿尔茨海默病诊断和预测的作用。
3. 缺失证据:文章没有给出足够充分的实验证据来支持作者所提出方法在实际应用中是否有效。
4. 宣传内容:文章过分宣传作者所提出方法在解决医学数据处理问题上具有优势,并未充分探讨其局限性和风险。
5. 偏袒:文章没有平等地呈现不同类型迁移学习方法之间的优缺点,而是过分强调作者所提出方法相对于其他方法具有优势。
6. 未探索反驳:文章没有探讨其他可能存在争议或反驳意见,并未充分考虑不同观点之间可能存在差异或冲突。
因此,在阅读本文时需要注意以上问题,并结合其他相关文献进行深入思考和评估。