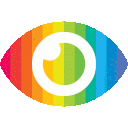
1. A novel method called PoinTr has been developed for point cloud completion, which reformulates the problem as a set-to-set translation task and adopts a Transformer encoder-decoder architecture.
2. PoinTr achieves promising performance with higher throughputs and fewer FLOPs compared to previous methods in practice.
3. The model also incorporates adaptive geometry-aware transformers, which enable diverse completion results by adapting to different geometric structures of input point clouds.
The article discusses a new method called PoinTr for point cloud completion, which reformulates the problem as a set-to-set translation task and uses a Transformer encoder-decoder architecture. The article claims that PoinTr achieves promising performance with higher throughputs and fewer FLOPs compared to previous methods.
Overall, the article appears to be well-written and informative. However, there are some potential biases and missing points of consideration that should be noted.
Firstly, the article does not provide much information about the dataset used for evaluating PoinTr's performance. It is unclear whether the dataset is representative of real-world scenarios or if it has any biases that could affect the results.
Secondly, while the article claims that PoinTr achieves better performance than previous methods, it does not provide detailed comparisons or statistical analyses to support this claim. Without such evidence, it is difficult to assess the validity of this claim.
Thirdly, the article does not explore any potential risks or limitations associated with using PoinTr for point cloud completion. For example, it is possible that PoinTr may struggle with certain types of point clouds or may produce inaccurate results in some cases.
Finally, there is a potential bias towards promoting PoinTr as a superior method for point cloud completion without fully exploring alternative approaches or counterarguments. While it is important to highlight new developments in research, it is also important to present a balanced view of different approaches and their strengths and weaknesses.
In conclusion, while the article provides useful information about PoinTr and its potential benefits for point cloud completion, there are some biases and missing points of consideration that should be taken into account when evaluating its claims.