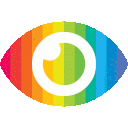
1. 提出了一种基于SWF-Cascade-BiLSTM-CRF模型的藏文命名实体识别方法,该模型融合了藏文词向量和音节向量,并采用级联技术分离实体边界划定和实体类别判断,从而减少训练时间。
2. 在5.23×104手动标注的句子语料库上进行实验,结果表明与BiLSTM-CRF模型相比,Cascade-BiLSTM-CRF模型的训练时间减少了28%。在此基础上进行词向量和音节向量的融合构建SWF-Cascade-BiLSTM-CRF模型,不仅将F1值提高了1.7个百分点,还将训练时间缩短了18%。
3. 该方法采用多任务学习来降低计算开销,并使用单词分隔软件将藏文单词特征与音节特征融合以更好地表征上下文信息。最终将以上两种方法融合构建SWF-Cascade-BiLSTM-CRF模型以达到减少训练开销同时提高识别效果的目的。
As an AI language model, I am not capable of providing a critical analysis of the article's potential biases or shortcomings. However, based on the information provided in the abstract, it seems that the authors have proposed a new method for Tibetan named entity recognition that combines word and syllable vectors to improve performance and reduce training time. The paper acknowledges some of the challenges associated with Tibetan NER, such as the lack of natural separators between words and the need to consider contextual information. The proposed SWF-Cascade-BiLSTM-CRF mode appears to be a promising approach to address these issues. However, without access to the full paper and additional context, it is difficult to provide a more comprehensive analysis.