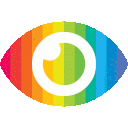
1. Islanding is a major issue in distributed generation (DG) where the DG continues to supply power into the distribution network even when the main utility power supply is interrupted.
2. A hybrid intelligent-based approach that combines passive and active techniques has been proposed for detecting islanding in synchronous machine-based DGs, using artificial neural networks (ANN) to process and analyze large data sets obtained from network simulations.
3. The proposed method uses a combination of passive parameters such as voltage, frequency, active and reactive power of DG, and total harmonic distortion of current, along with an active method that provides positive feedback via reactive power anti-islanding compensator to detect islanding operations with high accuracy.
The article presents a new hybrid intelligent-based approach for detecting islanding in distributed generation (DG). While the paper provides some useful information on the issue of islanding and the proposed technique, it has several limitations and biases that need to be addressed.
Firstly, the article lacks a comprehensive discussion of the potential risks associated with islanding operations of DGs. The authors only briefly mention that islanding can cause damages and dangers without providing any specific examples or statistics. This lack of information may lead readers to underestimate the severity of the problem.
Secondly, the article focuses solely on synchronous machine-based DGs and does not consider other types of DG sources such as static-inverter based generators. This narrow focus limits the applicability of the proposed method and ignores a significant portion of DG systems.
Thirdly, while the authors claim that their hybrid approach provides better reliability than existing techniques, they do not provide sufficient evidence to support this claim. The comparison with one reference is not enough to establish the superiority of their method over other techniques.
Fourthly, there is no discussion on potential drawbacks or limitations of using artificial neural networks (ANN) as a machine learning technology for processing and analyzing large data sets provided from network simulations. ANN may have limitations in terms of accuracy, interpretability, and generalizability.
Fifthly, the article does not address issues related to cost-effectiveness or practicality of implementing the proposed technique. The authors only mention that their method can secure detection for different network topology and various operating conditions but do not provide any information on how much it would cost to implement this technique in real-world scenarios.
Overall, while the proposed hybrid approach may have some potential benefits for detecting islanding in synchronous machine-based DGs, there are several limitations and biases in this article that need to be addressed before considering its practical application.