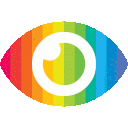
1. Indoor air quality in schools is important for providing a healthy learning environment, but particulate matter (PM10 and PM2.5) can exceed recommended limits and negatively impact student health.
2. Multivariate methods, such as multiple linear regression (MLR) and principal component regression (PCR), can be used to predict indoor PM10 and PM2.5 concentrations based on outdoor levels, meteorological factors, and building ventilation rates.
3. The study found that indoor PM10 and PM2.5 levels were affected by outdoor concentrations of these pollutants, as well as meteorological factors, and the developed models showed acceptable accuracy in predicting indoor concentrations.
The article titled "Multivariate methods for indoor PM10 and PM2.5 modelling in naturally ventilated schools buildings" provides an overview of the impact of particulate matter (PM10 and PM2.5) on indoor air quality in schools, with a focus on natural ventilation systems. The article highlights the potential health risks associated with exposure to high levels of PM, particularly among children who spend a significant amount of time indoors.
The article presents several studies that have found that the concentration of PM10 and PM2.5 in naturally ventilated school buildings often exceeds the limits recommended by the World Health Organization (WHO). The authors note that this is a particular concern in the Middle East region, where aerosol particle concentrations are influenced by several factors such as long-range aerosol transportation, seasonal dust storms, sea salt aerosol formation, and anthropogenic sources.
To address these concerns, the authors propose using multivariate statistical regression models to predict indoor concentrations of PM10 and PM2.5 based on various input parameters such as outdoor air pollutant levels, building ventilation rates, and meteorological parameters. The authors compare two types of regression models: multiple linear regression (MLR) and principal component regression (PCR).
While the article provides useful information about the potential health risks associated with exposure to high levels of PM in schools, there are several limitations to consider. Firstly, the article does not provide a comprehensive overview of all possible sources of indoor air pollution in schools. For example, other pollutants such as volatile organic compounds (VOCs) or carbon monoxide (CO) may also be present in indoor environments and could have an impact on student health.
Secondly, while the authors acknowledge that MLR can face serious difficulties when environmental variables are correlated with each other (multicollinearity), they do not provide a detailed explanation of how PCR addresses this issue or why it is a better approach than MLR.
Thirdly, while the authors note that deterministic models require a large amount of data as inputs and are difficult to find, they do not provide any evidence to support this claim or explain why MLR or PCR are more suitable alternatives.
Finally, while the authors acknowledge that their study was partly financed by Universiti Sains Malaysia (USM), they do not disclose any potential conflicts of interest or biases that may have influenced their research findings.
In conclusion, while this article provides some useful insights into the impact of particulate matter on indoor air quality in schools and proposes multivariate statistical regression models as a solution for predicting indoor concentrations of PM10 and PM2.5, there are several limitations to consider. These include incomplete coverage of all possible sources of indoor air pollution in schools, lack of detail regarding how PCR addresses multicollinearity issues compared to MLR, unsupported claims regarding deterministic models' data requirements being difficult to find without providing evidence for it; no disclosure about potential conflicts or biases influencing research findings.