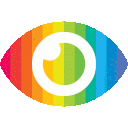
1. Cellular traffic prediction is becoming increasingly important for the success of modern communication systems, which support billions of mobile users and devices.
2. Artificial intelligence techniques, particularly machine learning and deep learning models, are being used to predict cellular traffic patterns and optimize network resource allocation.
3. The potential applications of cellular traffic prediction include base station sleeping, admission control, resource allocation and scheduling, network dimensioning, network slicing, software defined networking (SDN), and mobile edge computing.
The article "Cellular traffic prediction with machine learning: A survey" provides a comprehensive review of the relevant studies on cellular traffic prediction and classifies the prediction problems as temporal and spatiotemporal. The article also categorizes the prediction models with artificial intelligence into statistical, machine learning, and deep learning models and compares them. Various applications based on cellular traffic prediction are summarized along with their current progress, and potential research directions are pointed out for future research.
Overall, the article provides a thorough overview of cellular traffic prediction with machine learning techniques. However, there are some potential biases in the article that need to be considered. Firstly, the article mainly focuses on the use of artificial intelligence techniques for cellular traffic prediction, which may overlook other traditional methods that could be useful in certain scenarios. Secondly, while the article mentions various evaluation metrics used in different studies, it does not provide a critical analysis of these metrics or discuss their limitations.
Additionally, the article may have some one-sided reporting as it only presents studies that have been published from 2017 to 2021. This may exclude relevant studies conducted before this period that could provide valuable insights into cellular traffic prediction. Moreover, while the article discusses various potential applications based on cellular traffic prediction, it does not explore any possible risks associated with these applications.
Furthermore, there is no discussion of any counterarguments against using machine learning techniques for cellular traffic prediction or any potential drawbacks associated with these techniques. This lack of exploration may lead to an incomplete understanding of the topic.
In conclusion, while "Cellular traffic prediction with machine learning: A survey" provides a comprehensive overview of cellular traffic prediction using artificial intelligence techniques, it has some potential biases and limitations that need to be considered when interpreting its findings.