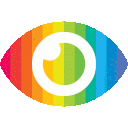
1. The paper explores identifying unique linguistic properties in texts written by humans versus those generated by large language models (LLMs), using hierarchical parse trees and recursive hypergraphs to uncover distinctive discourse patterns.
2. Empirical findings show that human-written texts exhibit more structural variability compared to machine-generated texts, reflecting the nuanced nature of human writing across different domains.
3. Incorporating hierarchical discourse features improves binary classifiers' ability to distinguish between human-written and machine-generated texts, even on out-of-distribution and paraphrased samples, highlighting the importance of analyzing text patterns at a deeper level.
The article "Threads of Subtlety: Detecting Machine-Generated Texts Through Discourse Motifs" explores the identification of unique linguistic properties in texts written by humans, with a focus on uncovering discourse structures beyond surface-level analysis. The study introduces a novel methodology that utilizes hierarchical parse trees and recursive hypergraphs to reveal distinctive discourse patterns in texts generated by both large language models (LLMs) and humans.
One potential bias in this article is the assumption that human-written texts inherently possess more structural variability compared to machine-generated texts. While the empirical findings suggest that human-written texts exhibit nuanced discourse patterns influenced by specific domains, it is important to consider that LLMs can also be trained on diverse datasets to mimic human-like writing styles. The article could benefit from a more nuanced discussion on the limitations of distinguishing between human and machine-generated texts based solely on discourse patterns.
Additionally, the article claims that incorporating hierarchical discourse features enhances binary classifiers' performance in distinguishing between human-written and machine-generated texts, even on out-of-distribution and paraphrased samples. However, there is a lack of detailed explanation or evidence provided to support this claim. It would be beneficial for the authors to include specific examples or case studies demonstrating how hierarchical discourse features improve classification accuracy.
Furthermore, the article mentions that the code and dataset will be made available at a later date, but does not provide any information on when or where they will be accessible. This lack of transparency could hinder reproducibility and further research in this area.
In terms of missing points of consideration, the article could delve deeper into potential ethical implications of detecting machine-generated texts. For example, how might this technology be used in misinformation detection or content moderation? Are there risks associated with relying too heavily on automated tools for text analysis?
Overall, while the article presents an interesting approach to identifying machine-generated texts through discourse motifs, there are areas where further clarification, evidence, and consideration of potential biases are needed to strengthen its argumentation and relevance in the field of natural language processing.