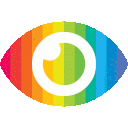
1. Researchers have developed a deep learning-based phenotyping approach that can accurately classify combined hepatocellular-cholangiocarcinoma (cHCC-CCA), a rare and aggressive liver cancer.
2. The deep learning model was trained on histopathological images of cHCC-CCA and other liver cancers, achieving high accuracy in distinguishing cHCC-CCA from other subtypes.
3. This approach has the potential to improve the diagnosis and classification of cHCC-CCA, leading to better treatment strategies for patients with this challenging disease.
The article titled "Deep learning-based phenotyping reclassifies combined hepatocellular-cholangiocarcinoma" published in Nature Communications presents a study on the classification of combined hepatocellular-cholangiocarcinoma (cHCC-CCA) using deep learning-based phenotyping. The authors aim to develop a reliable and accurate method for diagnosing cHCC-CCA, which is a rare and challenging liver cancer subtype.
The article provides detailed information about the methodology used in the study, including the collection of tissue samples from multiple centers, histopathological analysis, and the development of a deep learning algorithm for classification. The results show that the deep learning algorithm achieved high accuracy in distinguishing cHCC-CCA from other liver cancer subtypes.
While the study presents interesting findings and has potential clinical implications, there are several points that need to be critically analyzed. Firstly, the article does not provide sufficient information about potential biases in sample selection. It is unclear how representative the sample population is and whether there were any selection criteria that could introduce bias.
Additionally, there is limited discussion on potential limitations of the deep learning algorithm used in this study. Deep learning algorithms are known to be highly dependent on training data quality and quantity. It would have been valuable to discuss potential sources of error or misclassification that could affect the accuracy of the algorithm.
Furthermore, there is a lack of discussion on potential confounding factors or variables that could influence the classification accuracy. For example, it would have been interesting to explore whether patient demographics or clinical characteristics had any impact on the algorithm's performance.
The article also lacks a comprehensive discussion on alternative methods for diagnosing cHCC-CCA. While deep learning-based phenotyping shows promise, it would have been beneficial to compare its performance with other established diagnostic techniques such as immunohistochemistry or molecular profiling.
Moreover, there is no mention of potential risks or limitations associated with implementing this technology in clinical practice. Deep learning algorithms require large amounts of computational power and expertise, which may not be readily available in all healthcare settings. The feasibility and cost-effectiveness of implementing this technology should have been addressed.
Lastly, the article does not present both sides of the argument equally. While the study focuses on the potential benefits of deep learning-based phenotyping, it does not adequately discuss any limitations or challenges associated with this approach.
In conclusion, while the article presents interesting findings on the classification of cHCC-CCA using deep learning-based phenotyping, there are several critical points that need to be addressed. These include potential biases in sample selection, limitations of the algorithm used, confounding factors that could influence classification accuracy, alternative diagnostic methods, potential risks and limitations of implementation, and a balanced discussion on both the benefits and limitations of deep learning-based phenotyping.