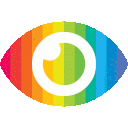
1. Non-profit organization LAION is building a dataset to create chatbots and AI models that understand human emotions and convert text into speech.
2. The 'Open Empathic' project aims to collect 10,000 audio samples initially, with a goal of reaching 100,000 to 1 million samples by next year.
3. Challenges in using AI to understand emotions include the difficulty of accurately reading people's emotions, biases in datasets, and potential misleading results in interpreting facial expressions.
The article discusses LAION, a non-profit organization that is building a dataset for artificial intelligence (AI) training to create chatbots that understand human emotions and AI models that convert text into speech. The project, called 'Open Empathic,' aims to go beyond understanding words and focus on identifying nuances of expressions and changes in tone to make human-AI interactions more realistic and empathetic.
While the article provides some information about the project, it lacks critical analysis and fails to address potential biases or limitations. It presents the project as a positive development without exploring potential risks or challenges associated with AI emotion recognition.
One-sided reporting is evident in the article's focus on the benefits of AI emotion recognition, such as its applications in driver fatigue detection, movie trailer assessment, job candidate screening, customer service, advertising evaluation, and mental health counseling. However, it fails to mention potential negative consequences or ethical concerns related to privacy invasion and manipulation of emotions.
The article also makes unsupported claims about the accuracy of AI emotion recognition technology. It states that "the general expectation is that it will only be a matter of time before you can detect the emotions of others on the phone or in a business meeting." However, there is no evidence provided to support this claim or any discussion about the limitations and challenges faced by current emotion recognition systems.
Furthermore, the article overlooks counterarguments regarding the difficulty of accurately reading human emotions. It briefly mentions that language and culture are not easy to read but does not delve into how these factors can impact emotion recognition. Different cultures have varying norms for expressing emotions, making it challenging for AI systems trained on specific datasets to generalize across diverse populations.
The article also fails to address biases in emotion detection datasets. It briefly mentions biases in open-source image datasets but does not explore how these biases can affect AI models' performance in recognizing emotions accurately. Additionally, it does not discuss potential biases introduced by human labelers when annotating emotional expressions in the dataset being built by LAION.
Overall, the article presents a one-sided and promotional view of AI emotion recognition without critically examining potential biases, limitations, or ethical concerns. It lacks evidence to support its claims and overlooks important considerations that should be taken into account when discussing AI emotion recognition technology.