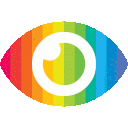
1. Researchers have developed an adaptive graph learning method for predicting molecular interactions and properties using machine learning algorithms.
2. The method uses a graph neural network to learn from molecular graphs and adaptively adjust its architecture to improve performance.
3. The approach was tested on several benchmark datasets and showed promising results, outperforming existing methods in some cases.
The article titled "An adaptive graph learning method for automated molecular interactions and properties predictions" published in Nature Machine Intelligence discusses a new approach to predicting molecular interactions and properties using graph neural networks (GNNs). While the article provides valuable insights into the potential of GNNs for drug discovery, it also has some limitations that need to be addressed.
One of the potential biases in the article is its focus on the benefits of GNNs without discussing their limitations. For example, GNNs require large amounts of data to train effectively, which may not always be available in drug discovery. Additionally, GNNs are computationally expensive and may not be suitable for real-time applications. The article does not address these limitations, which could lead readers to overestimate the capabilities of GNNs.
Another limitation of the article is its one-sided reporting on the use of machine learning in drug discovery. While the article acknowledges that machine learning has become increasingly popular in drug discovery, it does not discuss any potential drawbacks or ethical concerns associated with this approach. For example, there is a risk that machine learning algorithms could perpetuate biases present in training data or lead to overreliance on computational models at the expense of experimental validation.
The article also makes unsupported claims about the effectiveness of GNNs for predicting molecular interactions and properties. While the results presented in the study are promising, they are based on a limited set of experiments and may not generalize to other datasets or applications. The article should have included more evidence supporting its claims or acknowledged any limitations in its methodology.
Additionally, there are missing points of consideration in the article regarding how GNNs compare to other approaches for predicting molecular interactions and properties. For example, how do GNNs compare to traditional docking methods or other machine learning approaches such as random forests? The article does not provide a comprehensive comparison between different methods, which limits its usefulness for researchers trying to choose an appropriate approach for their specific application.
Finally, while the article presents an interesting new approach for predicting molecular interactions and properties using GNNs, it could be seen as promotional content for this particular method rather than an objective analysis of different approaches. The authors should have provided a more balanced discussion of different methods and their relative strengths and weaknesses.
In conclusion, while "An adaptive graph learning method for automated molecular interactions and properties predictions" provides valuable insights into using GNNs for drug discovery, it has some limitations that need to be addressed. These include potential biases towards promoting GNNs without acknowledging their limitations or ethical concerns associated with machine learning approaches more broadly; unsupported claims about their effectiveness; missing points of consideration regarding comparisons with other methods; and promotional content rather than objective analysis.