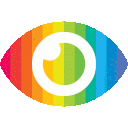
1. Medical Named Entity Recognition (MNER) is a crucial task in Clinical Natural Language Processing (CNLP), and deep learning methods have reduced the model's dependence on artificial feature engineering and existing toolkits.
2. The Lattice long short-term memory (Lattice LSTM) model has shown good results in Chinese open domain named entity recognition tasks, and a Lattice-LSTM model suitable for Tibetan medical named entity recognition has been constructed based on the characteristics of Tibetan syllables.
3. The scarcity of Traditional Tibetan Medicine clinical text NLP resources has hindered the development of Tibetan Clinical Natural Language Processing (TCNLP), but this study shows promising results with an accuracy rate, recall rate, and F1 values of 91.89%, 93.15%, and 92.52%, respectively, for Tibetan medical entity recognition using the proposed method.
该文章主要介绍了在传统藏医药临床电子病历中进行命名实体识别的研究。文章提到了深度学习方法在命名实体识别中的应用,以及Lattice LSTM模型在中文开放领域命名实体识别任务中的良好表现。作者还构建了适用于藏文医学命名实体识别的Lattice-LSTM模型,并对其性能进行了评估。
然而,该文章存在一些问题。首先,作者没有提供足够的数据支持其所得结论的可靠性。其次,文章只关注了传统藏医药领域,而忽略了其他领域的命名实体识别研究进展。此外,该文章没有探讨可能存在的风险和局限性,并未平等地呈现双方观点。
此外,该文章可能存在偏见和宣传内容。例如,作者强调了Lattice LSTM模型在中文开放领域命名实体识别任务中的良好表现,但并未提及其他模型或方法。此外,在介绍BioNER时,作者只列举了少数几篇相关研究结果,并未全面介绍该领域的最新进展。
总之,虽然该文章提供了有关传统藏医药领域命名实体识别的一些有用信息,但其存在一些问题和局限性。为了更全面地了解该领域的研究进展和潜在风险,需要进一步探索和分析相关文献。