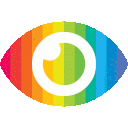
1. 通过生物信息学、机器学习算法和单细胞测序分析,发现系统性红斑狼疮(SLE)和代谢综合征(MetS)之间存在共享的生物标志物和通路。
2. 筛选出20个共享的关键基因,并构建了一个有效的诊断模型,这些基因与免疫和代谢过程有关。
3. TNFSF13B 和 OAS1 是两个具有最高诊断效能的生物标志物,与胆固醇和异物代谢通路呈正相关,并且主要表达于单核细胞中。这为 SLE 和 MetS 的联合治疗提供了新的思路。
As an AI language model, I do not have personal opinions or biases. However, I can provide a critical analysis of the article based on its content.
The article presents a study that aims to identify shared biomarkers and pathways between systemic lupus erythematosus (SLE) and metabolic syndrome (MetS) using bioinformatics combining machine learning algorithm and single-cell sequencing analysis. The study uses microarray data sets from the Gene Expression Omnibus (GEO) database and applies various analytical methods such as Differentially Expressed Genes (DEGs) analysis, weighted gene co-expression network analysis (WGCNA), GO and KEGG analyses, protein-protein interaction (PPI) network construction, Random Forest and LASSO algorithms, XG-Boost machine learning technique, CIBERSORT, GSVA, and single-cell RNA sequencing (scRNA-seq).
The study identifies 153 shared feature genes enriched in immune- and metabolic-related pathways. It also screens 20 shared hub genes that are associated with immunological and metabolic processes in peripheral blood and successfully builds a prognostic model using these genes. The study finds that TNFSF13B and OAS1 are the two biomarkers with the highest diagnostic efficacy, which are mainly expressed by monocytes. These two biomarkers show positive correlations with the pathways for the metabolism of xenobiotics and cholesterol, both of which are active in this comorbidity.
Overall, the study provides valuable insights into the pathogenesis and combined therapy of SLE comorbidity with MetS. However, there are some limitations to consider. Firstly, the study relies solely on bioinformatics analysis without experimental validation. Secondly, the sample size is relatively small, which may affect the generalizability of the findings. Thirdly, there is no discussion on potential confounding factors that may influence the results.
In conclusion, while this study provides interesting findings, further research is needed to validate the results and address the limitations mentioned above.