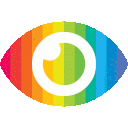
1. A hybrid wind speed prediction model based on Long Short-Term Memory (LSTM) network and improved BP neural network is proposed to deal with nonlinear wind speed prediction.
2. Singular spectrum analysis (SSA) and complete ensemble empirical model decomposition adaptive noise (CEEMDAN) are used as data pretreatment methods to de-noise the original wind speed data and decompose it into multiple components, respectively.
3. Fuzzy entropy (FE) is introduced to calculate the time complexity of each component, and an optimized BP neural network and LSTM are used to predict high complexity and low complexity subsequences, respectively, which are then superimposed to obtain the final values with higher prediction accuracy.
The article titled "Short-term wind speed forecasting based on long short-term memory and improved BP neural network" presents a novel combination forecasting model for wind speed prediction. The article provides a comprehensive review of the existing wind speed prediction models, including physical models, statistical models, artificial intelligence models, and hybrid models. The authors argue that the hybrid model is more comprehensive and can better highlight the functions required by a complete prediction system.
The article presents several contributions to the field of wind speed prediction. Firstly, it proposes using singular spectrum analysis (SSA) and complete ensemble empirical model decomposition adaptive noise (CEEMDAN) as data pretreatment methods to de-noise the original wind speed data and decompose it into multiple components. Secondly, fuzzy entropy (FE) is used to calculate the time complexity of each component, and according to Spearman correlation, the inherent mode function (IMF) components are recombined to form a new subsequence. Thirdly, two different prediction models are used to predict high complexity and low complexity subsequences separately.
Overall, the article provides a detailed description of the proposed method and its potential benefits in improving wind speed prediction accuracy. However, there are some limitations that need to be addressed. Firstly, while the authors provide a comprehensive review of existing wind speed prediction models, they do not discuss their limitations or potential biases. Secondly, there is no discussion on possible risks associated with using deep learning algorithms for wind speed prediction. Finally, there is no exploration of counterarguments or alternative approaches that could be used for wind speed prediction.
In conclusion, while the proposed method has potential benefits in improving wind speed prediction accuracy, further research is needed to address its limitations and potential biases. Additionally, it would be beneficial to explore alternative approaches that could complement or improve upon this method for more accurate predictions.