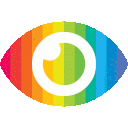
1. Medical concept embedding is crucial for healthcare analytics, but previous models do not consider the temporal nature of Electronic Medical Records (EMRs).
2. This article proposes a model that incorporates temporal information to embed medical codes by using the Continuous Bag-of-Words model and attention mechanism.
3. Experimental results show that this model outperforms five state-of-the-art baselines in clustering and nearest neighbor search tasks, demonstrating its effectiveness in capturing temporal correlations between medical concepts in EMRs.
The article titled "Medical Concept Embedding with Time-Aware Attention" proposes a model for embedding medical concepts in Electronic Medical Records (EMRs) that takes into account the temporal nature of the data. The authors argue that previous models that treat medical concepts and EMRs as words and documents respectively fail to capture the temporal correlations between medical concepts.
One potential bias in this article is the lack of discussion on potential limitations or drawbacks of incorporating temporal information into medical concept embedding. While the authors claim that their model outperforms five state-of-the-art baselines, they do not provide any evidence or analysis to support this claim. It would have been helpful to see a comparison of the performance metrics of their model against those of the baselines.
Additionally, the article does not address any potential risks or challenges associated with using their proposed model. For example, it is unclear how well their model would perform on large-scale datasets or how sensitive it is to variations in data quality. These considerations are important when evaluating the practicality and generalizability of a new approach.
Furthermore, there is no discussion on potential biases in the datasets used for evaluation. The article mentions using both public and proprietary datasets but does not provide any details about these datasets or how they were collected. This lack of transparency raises concerns about potential biases in the data and limits our ability to assess the generalizability of the findings.
Another limitation of this article is its narrow focus on clustering and nearest neighbor search tasks as measures of effectiveness. While these tasks can provide insights into the performance of a model, they do not necessarily capture all aspects relevant to healthcare analytics. It would have been beneficial to include additional evaluation metrics that assess other important aspects such as predictive accuracy or interpretability.
In conclusion, while the proposed model for medical concept embedding with time-aware attention is an interesting approach, this article lacks critical analysis and evidence to support its claims. The absence of discussions on limitations, potential risks, and biases in the datasets used raises concerns about the generalizability and practicality of the proposed model. Additionally, the narrow focus on specific evaluation tasks limits our understanding of its overall effectiveness in healthcare analytics.