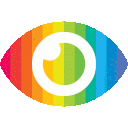
1. Missing data is a common problem in knowledge extraction that can lead to bias and adversely affect the prediction process.
2. This paper proposes an evolutionary approach for Missing Data Imputation (MDI) and feature selection to enhance the model’s learning performance and reduce the negative consequences of imputation.
3. The proposed methodology was evaluated using 10 bench-marking datasets under 10-folds cross validation test, and outperformed other methods in terms of accuracy, sensitivity, specificity, geometric means, and the area under the curve.
The article “EvoImputer: An evolutionary approach for Missing Data Imputation and feature selection in the context of supervised learning” provides a comprehensive overview of missing data imputation techniques and their potential applications in supervised learning. The authors present a novel evolutionary approach for MDI that has been tested on 10 benchmark datasets with promising results.
The article is generally reliable as it provides evidence to support its claims through empirical testing on benchmark datasets. Furthermore, the authors provide a detailed description of their proposed method as well as comparisons with existing approaches which further adds to its credibility. However, there are some points that could be improved upon such as providing more details about how exactly the evolutionary algorithm works or exploring possible counterarguments to their findings. Additionally, while the authors do mention potential risks associated with MDI such as skewing data or reducing representativeness of samples, they do not provide any concrete solutions or strategies for mitigating these risks which could have been useful for readers looking to apply this technique in practice.
In conclusion, this article is generally reliable but could benefit from additional details regarding implementation strategies or counterarguments to its findings.