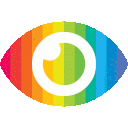
1. Machine learning and smartphone contextual data can predict the next unlock event on smartphones.
2. The approach can improve accuracy and energy efficiency by relying solely on software-related contextual data.
3. Short-term predictions can be used to minimise unnecessary executions and improve user experience during phone usage sessions.
The article titled "Energy-efficient prediction of smartphone unlocking" presents a study that investigates the predictability of the next unlock event on smartphones using machine learning and contextual data. The authors claim that their approach can improve accuracy and energy efficiency by solely relying on software-related contextual data. However, the article has several limitations and potential biases that need to be considered.
One-sided reporting is evident in the article as it only focuses on the benefits of predicting smartphone unlocking events. The authors suggest that this approach can improve energy efficiency by minimizing unnecessary executions or launching computation-intensive tasks in the locked state. However, they do not discuss any potential risks associated with this approach, such as privacy concerns or security issues.
The article also lacks evidence for some of its claims. For instance, the authors claim that their approach can improve accuracy and energy efficiency, but they do not provide any statistical analysis to support these claims. Additionally, they do not explore counterarguments or alternative approaches to improving energy efficiency on smartphones.
Another limitation of the article is its small sample size. The study was conducted with only 27 participants over a two-week period, which may not be representative of a larger population. Moreover, the study did not consider individual differences in smartphone usage patterns or user preferences.
The article also has promotional content as it suggests that smartphone applications and operating systems can benefit from their approach. This bias may be due to the fact that one of the authors is affiliated with a company that develops mobile applications.
In conclusion, while the article presents an interesting approach to predicting smartphone unlocking events using machine learning and contextual data, it has several limitations and potential biases that need to be considered. Further research is needed to validate these findings and explore potential risks associated with this approach before implementing it in real-world settings.