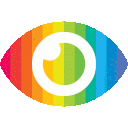
1. Object Detection and Tracking techniques have shown great potential in enhancing Precision Farming by providing real-time monitoring of crops and animals, optimizing resource allocation, and paving the way for robotization in the agricultural sector.
2. The integration of Deep Learning-based Object Detection with tracking offers advantages such as temporal continuity, object counting, trajectory analysis, and preservation of object identity, making it a robust approach for various applications in agriculture.
3. The review systematically analyzed over 300 research papers, highlighting the current advancements, challenges, and potential future research lines in implementing Computer Vision and Deep Learning methods within Precision Farming to maximize their impact on food security and sustainable agricultural practices.
The article "Object detection and tracking in Precision Farming: a systematic review" provides a comprehensive overview of the current literature on Object Detection and Tracking within the field of Precision Farming. The article highlights the importance of these techniques in enhancing Precision Farming by providing real-time monitoring of crops and animals, optimizing resource allocation, and paving the way for robotization in agriculture.
One potential bias in the article is the focus on the benefits and potential applications of Object Detection and Tracking in Precision Farming without adequately addressing potential risks or limitations. While the article mentions challenges faced by researchers, such as the lack of open-source datasets with labelled data, it does not delve deeply into other potential drawbacks or ethical considerations associated with implementing these technologies in agriculture.
Additionally, the article may have a slight bias towards promoting Deep Learning and Computer Vision techniques as the optimal solution for Object Detection and Tracking in Precision Farming. While these technologies have shown significant advancements in recent years, it is important to consider alternative approaches or methodologies that may also be effective in this context.
Furthermore, the article could benefit from exploring counterarguments or contrasting viewpoints regarding the integration of Object Detection and Tracking in Precision Farming. By presenting a more balanced perspective, readers would gain a more nuanced understanding of the complexities involved in implementing these technologies in agricultural practices.
Overall, while the article provides valuable insights into the current state of research on Object Detection and Tracking in Precision Farming, it could benefit from addressing potential biases, exploring alternative perspectives, and providing a more balanced discussion on both the benefits and challenges associated with these technologies.