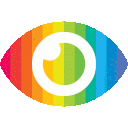
1. The article presents simCAS, an embedding-based simulator for generating high-fidelity single-cell chromatin accessibility sequencing (scCAS) data.
2. simCAS outperforms existing simulators in resembling real data and can generate cells of different states with user-defined cell populations and differentiation trajectories.
3. simCAS facilitates the benchmarking of four core tasks in downstream analysis: cell clustering, trajectory inference, data integration, and cis-regulatory interaction inference.
The article titled "simCAS: an embedding-based method for simulating single-cell chromatin accessibility sequencing data" presents a new simulator for generating synthetic data from single-cell chromatin accessibility sequencing (scCAS) technology. The authors argue that existing simulators are inadequate in providing credible ground-truth labels for method evaluation, and their proposed simCAS outperforms them in resembling real data.
The article provides a detailed description of the simCAS simulator, including its ability to generate high-fidelity scCAS data from both cell-wise and peak-wise embeddings. The authors demonstrate that simCAS can generate cells of different states with user-defined cell populations and differentiation trajectories, simulate data from different batches, and encode user-specified interactions of chromatin regions in the synthetic data.
The authors systematically demonstrate that simCAS facilitates the benchmarking of four core tasks in downstream analysis: cell clustering, trajectory inference, data integration, and cis-regulatory interaction inference. They anticipate that simCAS will be a reliable and flexible simulator for evaluating ongoing computational methods applied on scCAS data.
Overall, the article appears to be well-written and informative. However, there are some potential biases and limitations to consider. Firstly, the authors do not provide any information about their funding sources or potential conflicts of interest. This lack of transparency could raise concerns about the objectivity of their research.
Secondly, while the authors claim that simCAS outperforms existing simulators in resembling real data, they do not provide any evidence to support this claim beyond visual comparisons. It would be helpful if they could provide more quantitative measures or statistical tests to validate their claims.
Thirdly, while the authors demonstrate how simCAS can facilitate benchmarking of downstream analysis tasks, they do not explore any potential limitations or challenges associated with using synthetic data for evaluation purposes. For example, it is unclear how well synthetic data generated by simCAS would generalize to real-world scenarios or whether it could introduce biases or artifacts that could affect downstream analysis results.
Finally, the article appears to be promotional in nature, with the authors emphasizing the advantages of their proposed simCAS simulator without discussing any potential drawbacks or limitations. It would be helpful if they could provide a more balanced perspective on the strengths and weaknesses of their approach.
In conclusion, while the article presents an interesting new simulator for generating synthetic scCAS data, there are some potential biases and limitations to consider. Future research should aim to address these issues and provide a more comprehensive evaluation of simCAS's performance and utility.