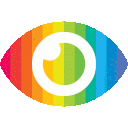
1. This protocol describes a framework that integrates the design of sustainable waste-to-energy processes and the optimization of operating parameters using AI tools.
2. The framework can be applied to various types of waste, such as medical waste, sewage sludge, plastic waste, and food waste.
3. By implementing this framework, insights into economically viable and carbon-neutral waste valorization can be obtained, contributing to more sustainable waste management practices and climate change mitigation efforts.
The article titled "Protocol for the design and accelerated optimization of a waste-to-energy system using AI tools" provides a framework for designing and optimizing waste-to-energy systems using artificial intelligence (AI) tools. The article discusses the integration of sustainable waste conversion processes with high-efficiency operating parameters and highlights the case of converting medical waste into methanol. It also mentions that the framework can be adapted to other types of waste such as sewage sludge, plastic waste, and food waste.
One potential bias in the article is its focus on the positive aspects of waste-to-energy systems without adequately addressing potential negative impacts or risks. While it mentions that the framework aligns with global efforts to reduce greenhouse gas emissions and transition to a low-carbon economy, it does not discuss any potential environmental or health concerns associated with waste incineration or other waste conversion processes. This one-sided reporting may give readers an incomplete understanding of the overall sustainability and feasibility of waste-to-energy systems.
Additionally, the article lacks evidence or data to support some of its claims. For example, it states that through the implementation of this framework, new insights into waste valorization with economic viability and carbon neutrality can be obtained. However, no specific examples or studies are provided to support this claim. Without supporting evidence, readers may question the validity and reliability of these statements.
Furthermore, the article does not explore counterarguments or alternative perspectives on waste management practices. It presents waste-to-energy systems as a significant step towards sustainable waste management without discussing potential alternatives such as recycling or reducing waste generation at the source. By not presenting a balanced view, the article may be promoting a specific solution without considering other viable options.
The promotional nature of the article is evident in its discussion of specific software packages for process simulation and optimization. While it briefly mentions open-source software like DWSIM, most of the listed software packages are commercial products with expensive licensing fees. This emphasis on commercial software may give the impression that these tools are necessary for implementing the proposed framework, potentially excluding readers who do not have access to or cannot afford such software.
Overall, the article provides a framework for designing and optimizing waste-to-energy systems using AI tools but lacks a balanced discussion of potential risks, alternative approaches, and supporting evidence for its claims. Its focus on commercial software and lack of consideration for open-source alternatives may also introduce bias. Readers should approach the information presented critically and seek additional sources to gain a comprehensive understanding of waste management practices.